Events
Upcoming Events
There are no upcoming events matching your criteria.
Past Events
National Academies Digital Twin Workshops - Atmospheric, Climate, and Sustainability Science
Wednesday, Feb. 1, 2023, 9 a.m. through Thursday, Feb. 2, 2023, 9 a.m.
Virtual
A National Academies of Sciences, Engineering, and Medicine-appointed ad hoc committee will identify needs and opportunities to advance the mathematical, statistical, and computational foundations of digital twins in applications across science, medicine, engineering, and society. They have organized a series of workshops including this workshop focusing on focusing on atmospheric, climate, and sustainability science.
This workshop invites speakers and panelists—including Mike Goodchild (University of California - Santa Barbara), Venkatramani Balaji (Schmidt Futures), Amy McGovern (University of Oklahoma), Anima Anandkumar (California Institute of Technology), and others—to discuss the definition of a digital twin and its applications.
The workshop will focus on key technical challenges for developing and using digital twins, including issues related to ML/AI, big data, and data assimilation. The workshop will also explore opportunities for translation of promising practices to and from other fields.
Register for the project's information-gathering workshop on digital twins in the Atmospheric, Climate, and Sustainability Science field.
February 1, 9:00 AM - 1:30 PM (CST) and February 2, 9:00 AM - 12:00 PM (CST)
National Academies Digital Twin Workshops - Biomedical
Monday, Jan. 30, 2023, 1 p.m.
Virtual via registration.
A National Academies of Sciences, Engineering, and Medicine-appointed ad hoc committee will identify needs and opportunities to advance the mathematical, statistical, and computational foundations of digital twins in applications across science, medicine, engineering, and society.
Register for the project's information-gathering workshop on digital twins in the Biomedical field (January 30).
For more information, please visit the National Academies website.
Machine Learning Seminar Series with Yifan Peng (PHS, Cornell U.)
Wednesday, Dec. 14, 2022, 11 a.m.
Hybrid Event:
3-180 Keller Hall
Join the Zoom
Clinical natural language processing and deep learning in assisting medical image analysis
Medical imaging has been a common examination in daily clinical routines for screening and diagnosis of a variety of diseases. Although hospitals have accumulated a large number of image exams and associated reports, it is yet challenging to use them to build high-precision computer-aided diagnosis systems effectively. In this talk, I will present an overview of cutting-edge techniques for mining existing free-text report data to assist medical image analysis via natural language processing and deep learning. Specifically, I will discuss both pattern-based and machine learning-based methods to detect findings/diseases and their attributes (e.g., type, location, size) from the chest x-ray and CT reports. Using these methods, we can construct large-scale medical image datasets with rich information. I will also demonstrate three case studies of medical image analysis using these datasets: (i) common thorax disease detection and report generation from chest X-rays and (ii) lesion detection and segmentation from CT images.
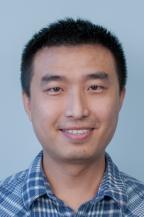
About Dr. Peng
Dr. Peng is an assistant professor at the Department of Population Health Sciences at Weill Cornell Medicine. His main research interests include BioNLP and medical image analysis. Before joining Cornell Medicine, Dr. Peng was a research fellow at the National Center for Biotechnology Information (NCBI), the National Library of Medicine (NLM), National Institutes of Health (NIH). He obtained his Ph.D. degree from the University of Delaware. During his doctoral training, he investigated applications of machine learning in biomedical text-mining, with a focus on deep analysis of the linguistic structures of biomedical texts.
Computer Science Colloquium: On Leaky Models and Unintended Inferences
Monday, Dec. 12, 2022, 11:15 a.m.
2-230 Kenneth H. Keller Hall
David Evans (University of Virginia)
Machine learning offers the promise to train models that perform surprisingly well on a wide range of tasks, merely by using massive computing power and generic training algorithms on available data sets. It is an open question, however, what else those models might learn about their training data, and how an adversary with some access to the model may be able to reveal it.
In this talk, I will discuss a variety of inference risks associated with machine-trained models, with a particular focus on surprising (and potentially harmful) things a model may reveal not just about individual training records but about the overall distribution of its training data. This includes attacks an adversary may use to learn statistical properties about the training distribution and about whether certain kinds of data are or are not included, and the potential for an adversary to use a model to make sensitive inferences about individuals, even for attributes not directly related to the task and regardless of whether those individuals are included the training data.
I’ll conclude with some thoughts on why defending against these types of attacks is hard, and what we might learn about how we should be training and exposing models.
About David Evans
David Evans is a Professor of Computer Science at the University of Virginia where he leads research on security and privacy with a recent focus on adversarial machine learning and inference risks in machine learning, and teaches courses on a wide variety of topics including biology, ethics, economics, and theory of computing. He is the author of an open computer science textbook and a children's book on combinatorics and computability and co-author of a book on secure computation.
He won the Outstanding Faculty Award from the State Council of Higher Education for Virginia and is Program Co-Chair for the 2022 and 2023 IEEE European Symposia on Security and Privacy. He was Program Co-Chair for the 24th ACM Conference on Computer and Communications Security (CCS 2017) and the 30th (2009) and 31st (2010) IEEE Symposia on Security and Privacy, where he initiated the Systematization of Knowledge (SoK) papers.
He has SB, SM and PhD degrees in Computer Science from MIT and has been a faculty member at the University of Virginia since 1999.
IMA Industrial Problems Seminar: Data Science to Software Engineering and Back Again
Friday, Dec. 9, 2022, 1:25 p.m.
Registration is required to access the Zoom webinar.
Cora Brown (Bridge Financial Technology)
In this talk, I will discuss my early career as a Data Scientist and Software Engineer. The skills necessary for these two types of roles overlap and complement each other. Drawing on my experiences in both fields, I will share some of the skills I’ve found valuable in each position and why I’ve chosen to follow this path. I will focus on the ways in which developing solid software skills have made me a better Data Scientist. Finally, I will describe some of the specific problems I’ve worked on as a Data Scientist and Software Engineer and how a background in mathematics can aid in solving these problems.
View the IMA event page
Machine Learning Seminar Series with Zhengyuan Zhou (Stern School of Business, NYU)
Wednesday, Dec. 7, 2022, 11 a.m.
Hybrid Event:
3-180 Keller Hall
Join the Zoom
Optimal No-Regret Learning in Repeated First-Price Auctions
First-price auctions have very recently swept the online advertising industry, replacing second-price auctions as the predominant auction mechanism on many platforms for display ads bidding. This shift has brought forth important challenges for a bidder: how should one bid in a first-price auction, where unlike in second-price auctions, it is no longer optimal to bid one's private value truthfully and hard to know the others' bidding behaviors?
In this talk, we take an online learning angle and address the fundamental problem of learning to bid in repeated first-price auctions. We discuss our recent work in leveraging the special structures of the first-price auctions to design minimax optimal no-regret bidding algorithms.
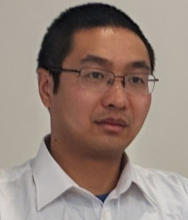
About Zhengyuan Zhou
Zhengyuan Zhou is currently an assistant professor in New York University Stern School of Business, Department of Technology, Operations and Statistics. Before joining NYU Stern, Professor Zhou spent the year 2019-2020 as a Goldstine research fellow at IBM research. He received his BA in Mathematics and BS in Electrical Engineering and Computer Sciences, both from UC Berkeley, and subsequently a PhD in Electrical Engineering from Stanford University in 2019. His research interests lie at the intersection of machine learning, stochastic optimization and game theory and focus on leveraging tools from those fields to develop methodological frameworks to solve data-driven decision-making problems.
Data Science Poster Fair
Friday, Dec. 2, 2022, 10 a.m.
Keller H. Hall 3-176 and Keller Atrium
The department of Computer Science and Engineering invites you to attend the annual Data Science Poster Fair!
As a part of their degree requirements, Data Science M.S. students conduct research under the direction of a faculty advisor culminating with a capstone project and poster presentation. Students presenting at this event will discuss details of their capstone project, including their driving question, methods, and results, and will be available to address questions and connect with guests during their time slot. Faculty from numerous departments across the University are affiliated with the data science graduate program, therefore a variety of areas are represented with each student’s capstone project.
The poster fair is open to the public and all interested undergraduate and graduate students, alumni, staff, faculty, and industry professionals are encouraged to attend.
View the CS&E event page for the poster information
Machine Learning Seminar Series with Xiaoran Sun (FSS, CEHD, UMN)
Wednesday, Nov. 30, 2022, 11 a.m.
Hybrid Event:
3-180 Keller Hall
Join the Zoom
Machine Learning for Human Development and Family Research: An Overview and an Example
This talk will first provide a brief overview about the utility of machine learning (ML) in research on developmental and family science by presenting what ML can offer in the face of theories and research questions in this field. Then the talk will introduce a study using a literature-driven supervised ML approach for empirical synthesis on how family experiences during adolescence predict future educational outcomes in adulthood. Based on the utility and the empirical synthesis example, there will be a discussion about future steps for how we can expand on the use of ML in social science research. Note that this talk will be focused on the applications of ML instead of technical details of advancing ML itself. Questions, discussions, and comments will all be super appreciated given the project is still in its development stage.
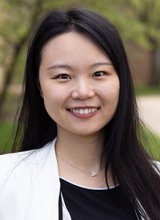
About Xiaoran Sun
Xiaoran Sun is an assistant professor in the Department of Family Social Science at the University of Minnesota. She is also a faculty affiliate of the Learning Informatics Lab in the College of Education and Human Development and of the Data Science Initiative. She obtained her PhD in Human Development and Family Studies from the Pennsylvania State University with an NSF traineeship on Big Data Social Science. Before joining UMN she was a postdoctoral scholar at Stanford University in the Departments of Pediatrics and Communication and a Stanford Data Science scholar. She uses ML in her research on family systems and adolescent development.
IMA Data Science Seminar: Benefits of Weighted Training in Machine Learning and PDE-based Inverse Problems
Tuesday, Nov. 29, 2022, 1:25 p.m.
Registration is required to access the Zoom webinar.
Yunan Yang (ETH Zürich)
Many models in machine learning and PDE-based inverse problems exhibit intrinsic spectral properties, which have been used to explain the generalization capacity and the ill-posedness of such problems.
In this talk, we discuss weighted training for computational learning and inversion with noisy data. The highlight of the proposed framework is that we allow weighting in both the parameter space and the data space. The weighting scheme encodes both a priori knowledge of the object to be learned and a strategy to weight the contribution of training data in the loss function. We demonstrate that appropriate weighting from prior knowledge can improve the generalization capability of the learned model in both machine learning and PDE-based inverse problems.
View the IMA event page
IMA Data Science Seminar: Probabilistic Inference on Manifolds and Its Applications in 3D Vision
Tuesday, Nov. 22, 2022, 1:25 p.m.
Registration is required to access the Zoom webinar.
Tolga Birdal (Imperial College London)
Stochastic differential equations have lied at the heart of Bayesian inference even before being popularized by the recent diffusion models. Different discretizations corresponding to different MCMC implementations have been useful in sampling from non-convex posteriors. Through a series of papers, Tolga and friends have demonstrated that this family of methods are well applicable to the geometric problems arising in 3D computer vision.
Before inviting the rest of this community to geometric diffusion models, Tolga will share his perspectives on two topics: (i) foundational tool of Riemannian MCMC methods for geometric inference and (ii) applications in probabilistic multi view pose estimation as well as inference of combinatorial entities such as correspondences. If time permits, Tolga will continue with his explorations in optimal-transport driven non-parametric methods for inference on Riemannian manifolds.
Relevant papers include:
- Bayesian Pose Graph Optimization [NeurIPS 2018]
- Pobabilistic Permutation Synchronization [CVPR 2019 Honorable Mention]
- Synchronizing Probability Measures on Rotations [CVPR 2020]
View the IMA event page