Winter Math-to-Industry Boot Camp
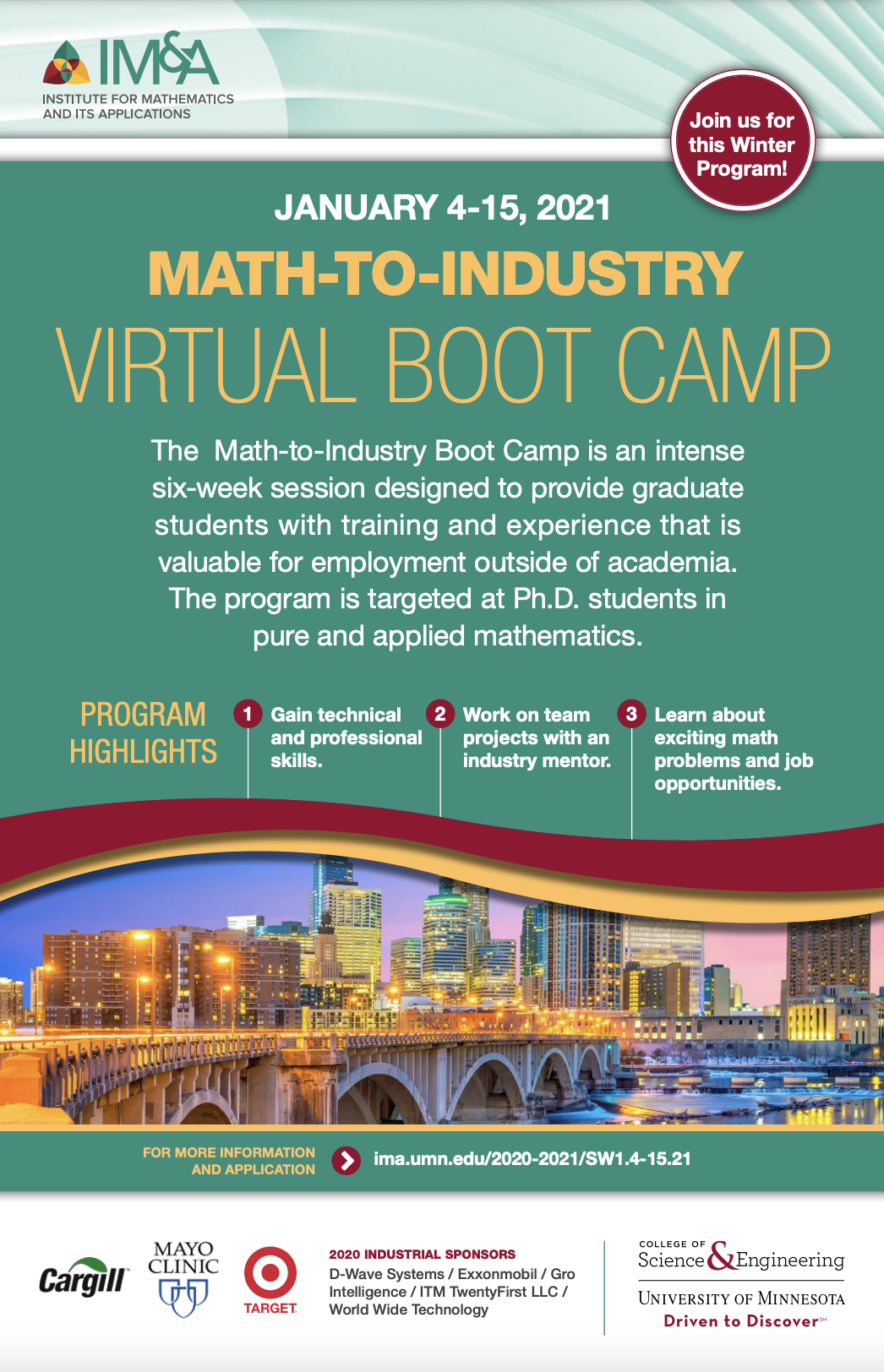
Advisory: Application deadline is Friday, December 4, 2020
2021 Winter Virtual Boot Camp poster
Organizers:
- Jasmine Foo, University of Minnesota, Twin Cities
- Thomas Hoft, University of St. Thomas
- Daniel Spirn, University of Minnesota, Twin Cities
The Winter Math-to-Industry Boot Camp is an intensive, two-week program that provides graduate students with training and experience that is valuable for employment outside of academia. The program is targeted at Ph.D. students in mathematics and statistics. The winter camp consists of pre-camp coursework in the basics of programming, data analysis, and optimization.
During the program, students work in small teams under the guidance of an industry mentor using a variety of streaming technology. The mentor and camp staff will help guide the students in the modeling process, analysis, and computational work associated with a real-world industrial problem. Additional time will be spent on developing professional and networking skills, meeting industry scientists, and participating in a career fair.
Each team will be expected to make a final presentation and submit a written report at the end of the workshop.
Recent industrial sponsors included Cargill, D-Wave Systems, the Mayo Clinic, Securian Financial, World Wide Technology.
Eligibility
Applicants must be current graduate students in a mathematical sciences Ph.D. program at a U.S. institution during the period of the boot camp.
Logistics
The program will take place online. Students will receive a $500 stipend.
Applications
To apply, please supply the following materials through the link at the top of the page:
- Statement of reason for participation, career goals, and relevant experience
- Unofficial transcript, evidence of good standing, and have full-time status
- Letter of support from advisor, director of graduate studies, or department chair
Selection criteria will be based on background and statement of interest, as well as geographic and institutional diversity. Women and minorities are especially encouraged to apply. Selected participants will be contacted in December.
Participants
Name | Department | Affiliation |
---|---|---|
Daniel Alhassan | Department of Mathematics and Statistics | Missouri University of Science and Technology |
Mohamed Imad Bakhira | Department of Mathematics | The University of Iowa |
Yiqing Cai | Gro Intelligence | |
Frankie Chan | Department of Mathematics | Purdue University |
Jorge Cisneros Paz | Department of Applied Mathematics | University of Washington |
Paula Dassbach | Medtronic | |
Jerry Dogbey-Gakpetor | Statistics | North Dakota State University |
Henry Fender | Department of Data Science | ITM TwentyFirst LLC |
Shihang Feng | Applied Mathematics and Plasma Physics | Los Alamos National Laboratory |
Jasmine Foo | School of Mathematics | University of Minnesota, Twin Cities |
Jonathan Hill | ITM TwentyFirst LLC | |
Thomas Hoft | Department of Mathematics | University of St. Thomas |
Salomea Jankovic | Department of Mathematics | University of Minnesota, Twin Cities |
Henry Kvinge | Pacific Northwest National Laboratory | |
Axel La Salle | School of Mathematical and Statistical Sciences | Arizona State University |
Youzuo Lin | Earth and Environmental Sciences Division | Los Alamos National Laboratory |
Sander Mack-Crane | Department of Mathematics | University of California, Berkeley |
Maia Powell | Department of Applied Mathematics | University of California, Merced |
Lee Przybylski | Mathematics | Iowa State University |
Priyanka Rao | Department of Mathematics & Statistics | Washington State University |
Majerle Reeves | Department of Applied Mathematics | University of California, Merced |
Daniel Spirn | University of Minnesota | University of Minnesota, Twin Cities |
Anna Srapionyan | Merrill Lynch | |
Wencel Valega Mackenzie | Department of Mathematics | University of Tennessee |
Christine Vaughan | Department of Mathematics and Mechanical Engineering | Iowa State University |
Elise Walker | Department of Mathematics | Texas A & M University |
Max Wimberley | Department of Mathematics | University of California, Berkeley |
Harrison Wong | Department of Mathematics | Purdue University |
Cancan Zhang | Department of Mathematics | Northeastern University |
Projects and teams
Project 1: Record Linkage: Synthesizing Expert Systems and Machine Learning
- Mentor Jonathan Hill, ITM TwentyFirst LLC
- Mentor Henry Fender, ITM TwentyFirst LLC
- Jorge Cisneros Paz, University of Washington
- Jerry Dogbey-Gakpetor, North Dakota State University
- Majerle Reeves, University of California, Merced
- Elise Walker, Texas A & M University
- Max Wimberley, University of California, Berkeley
- Harrison Wong, Purdue University
Record linkage is a common big data process where shared records in two large datasets are linked based on common fields. Longevity Holdings designed an expert system to automate record linkage between client data and a corpus of death records. This system produces scores that sort record pairs into matches and non-matches. Currently, high and low scores separate cleanly, but mid-tier scores must be manually reviewed. This led us to ask: Can machine learning improve an expert system in record linkage and reduce the size of this review set?
We are working with a variant of the Expectation Maximization (EM) algorithm following the Fellegi-Sunter approach to record linkage. We implemented this algorithm but have not found an optimal configuration for our data. The algorithm is general so we can manipulate many aspects of the input. Our priority is to determine whether there is a configuration that can improve the expert system.
EM is not the only viable approach to this problem. There are a wide range of existing methods that can be applied to record linkage. Our priority is to figure out the pros and cons for each, while trying to exceed EM and expert system performance.
On this project, you will work with real-world data and learn to organize as a team. You will deliver a whitepaper summarizing your process and results. We are most interested in your clear thinking and structured approach to this problem. We will divide into two groups focusing on one of the priorities above. Both groups will receive two validated sets of record pairs, one deriving from obituaries and the other from state and federal records. Our toolset will include python, pandas, and scikit-learn.
Project 2: Data-Driven Computational Seismic Inversion
- Mentor Youzuo Lin, Los Alamos National Laboratory
- Mentor Shihang Feng, Los Alamos National Laboratory
- Frankie Chan, Purdue University
- Salomea Jankovic, University of Minnesota, Twin Cities
- Sander Mack-Crane, University of California, Berkeley
- Priyanka Rao, Washington State University
- Christine Vaughan, Iowa State University
- Cancan Zhang, Northeastern University
Computational seismic inversion turns geophysical data into actionable information. The technique has been widely used in geophysical exploration to characterize the subsurface structure. Such a clear and accurate map of the subsurface is crucial for determining the location and size of reservoirs and mineral features.
Seismic inversion usually presents itself as an inverse problem. However, solving those inverse problems has been notoriously challenging due to their ill-posed and computationally expensive nature. On the other hand, with advances in machine learning and computing, and the availability of more and better data, there has been notable progress in solving such problems. In our recent work [1, 2], we developed end-to-end data-driven subsurface imaging techniques and produced encouraging results when test data and training data share similar statistics characteristics. The high accuracy of the predictive model is built on the assumption that the training dataset captures the distribution of the target dataset. Therefore, it is critical to obtain a sufficient amount of high-quality training set.
In this project, students will work with LANL scientists to study the impact of the training data on the resulting predictive model. In particular, students will explore and develop different techniques to generate high-quality synthetic data that could be used to enhance the training data quality. Through the project, students will have the opportunity to learn deep learning and its applications in computational imaging and the fundamentals of ill-posed inverse problems.
Reference:
[1]. Yue Wu and Youzuo Lin, “InversionNet: An Efficient and Accurate Data-driven Full Waveform Inversion,” IEEE Transactions on Computational Imaging, 6(1):419-433, 2019.
[2]. Zhongping Zhang and Youzuo Lin, “Data-driven Seismic Waveform Inversion: A Study on the Robustness and Generalization,” in IEEE Transactions on Geoscience and Remote Sensing, 58(10):6900-6913, 2020.
Project 3: The Impact of Climate Change on Crop Yield
- Mentor Yiqing Cai, Gro Intelligence
- Daniel Alhassan, Missouri University of Science and Technology
- Mohamed Imad Bakhira, The University of Iowa
- Axel La Salle, Arizona State University
- Maia Powell, University of California, Merced
- Lee Przybylski, Iowa State University
- Wencel Valega Mackenzie, University of Tennessee
Gro is a data platform with comprehensive data sources related to food and agriculture. With data from Gro, stakeholders can make quicker and better decisions. In this project, the students will use data from Gro to quantify the impact of climate change on crop yield, and create visualizations to demonstrate their findings. For example, they can use long-term climate data from Gro, to predict corn yield in Minnesota, 100 years from now. Based on the results, they might be able to conclude that Minnesota will no longer be suitable for growing corn in 100 years, or the areas suitable for corn will shift from the south to the north within Minnesota. Furthermore, they can scale the analysis to the whole globe, and create cool visualizations to show the results.
Data will be provided through Gro API (Python client). For data discovery and visualizations, the students can interact with the Gro web app directly. Once they decide what data to pull from Gro, they can export a code snippet and use the API client to download the data. Data pulled from Gro are in the format of time series, which are called data series. A data series is made up of data points, each with a start and end timestamp. Different data series can come from different sources, and have different frequencies. For example, there are projected monthly precipitation and air temperature from the GFDL B1 model all the way to year 2100, that are available across the whole world.
The deliverables of this project are two-fold: a Jupyter notebook (hosted on Infrastructure provided by Gro) and a visual presentation of the results. It can even be the combination of the two. The Jupyter notebook should be executable end-to-end, from fetching the data from Gro API, to export predictions as files, or as visualizations.