ISyE Seminar Series: Graduate Showcase
"Single Sample Estimation from Cancer Recurrence Data"
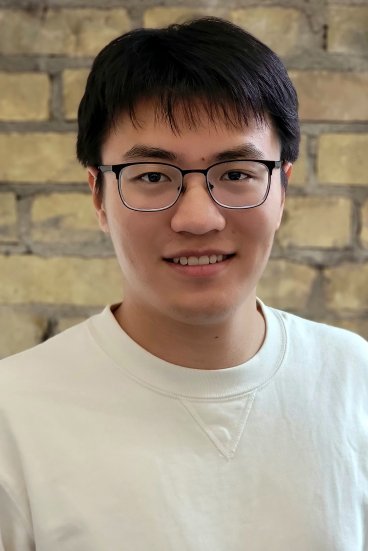
Xuanming Zhang
Ph.D Candidate
University of Minnesota
About the seminar:
In this study, we aim to develop consistent estimators for crucial parameters that regulate the dynamics of tumor cell populations under drug treatment. While drug therapy initially reduces the number of drug-sensitive cells, a population of drug-resistant cells eventually emerges, leading to a resurgence of the tumor burden. To accomplish this, we will derive several large number limit theorems, specifically focusing on the metrics that quantify clonal diversity at the point of cancer recurrence. These theorems will serve as the foundation for constructing consistent estimators.
Bio:
Xuanming Zhang is a third-year PhD candidate in the Department of Industrial and Systems Engineering at the University of Minnesota. He has the privilege of being advised by Dr. Kevin Leder. Zhang's research primarily focuses on the mathematical modeling of cancer evolution and tumor therapy, and his areas of interest encompass stochastic processes, cancer evolution, and reinforcement learning."
"Markov Persuasion Processes with Endogenous Agent Beliefs"

You Zu
Ph.D Candidate
University of Minnesota
About the seminar:
We consider a dynamic Bayesian persuasion setting where a single long-lived sender persuades a stream of ``short-lived'' agents (receivers) by sharing information about a payoff-relevant state. The state transitions are Markovian conditional on the receivers' actions, and the sender seeks to maximize the long-run average reward by committing to a (possibly history-dependent) signaling mechanism. Such problems are common in platform markets, where the platform seeks to achieve desirable long-term revenue and welfare outcomes by influencing the actions of users. While most previous studies of Markov persuasion consider exogenous agent beliefs that are independent of the chain, we study a more natural variant with \emph{endogenous} agent beliefs that depend on the chain's realized history. A key challenge to analyzing such settings is to model the agents' partial knowledge about the history information. To address this challenge, we analyze a Markov persuasion process (MPP) under various information models that differ in the amount of information the receivers have about the history of the process. Specifically, we formulate a general partial-information model where each receiver observes the history with an $\ell$ periods lag (for $\ell \geq 0)$. Our technical contribution starts with analyzing two benchmark models, i.e., the full-history information model (i.e., $\ell = 0$) and the no-history information model (i.e., $\ell = \infty$). We establish an ordering of the sender's payoff as a function of the informativeness of the agent's information model (with no-history as the least informative), and develop efficient algorithms to compute optimal solutions for these two benchmarks. For the information model with general $\ell$, we present the technical challenges in finding an optimal signaling mechanism, where even determining the right dependency on the history becomes difficult. Restricting the dependence on the history to a given length, we formulate the sender's problem as a bilinear optimization program. To bypass the resulting computational complexity, we use a robustness framework to design a ``simple'' \emph{history-independent} signaling mechanism that approximately achieves optimal payoff when $\ell$ is reasonably large.
Bio:
You Zu is a fifth-year Ph.D. student in Industrial Systems and Engineering. Her research interests include information design, algorithmic game theory, and data science. Her current research focuses on optimal information sharing in dynamic environments.
"Using Birth-death Processes to Infer Tumor Subpopulation Structure from Live-cell Imaging Drug Screening Data"

Chenyu Wu
Ph.D Candidate
University of Minnesota
About the seminar:
Tumor heterogeneity is a complex trait that poses significant challenges in the development of effective cancer therapies. Accurately characterizing the subpopulation structure within a tumor is crucial. One possible strategy is to analyze the differential responses of subpopulations to various drugs using live-cell imaging high throughput drug screen techniques. However, such data exhibits dynamic variance and positive correlation in time, making analysis difficult.To address this challenge, we propose a stochastic model based on the linear birth-death process. Our newly developed model can provide more precise and robust estimations than the existing methods. We conclude our study by testing our model on both simulated data (in silico) and experimental data (in vitro), which supports our argument about its advantages.
Bio:
Chenyu Wu is a 4th year Ph.D. student at the ISyE department at the University of Minnesota. He is working with Dr. Kevin Leder.