Welcoming new faculty
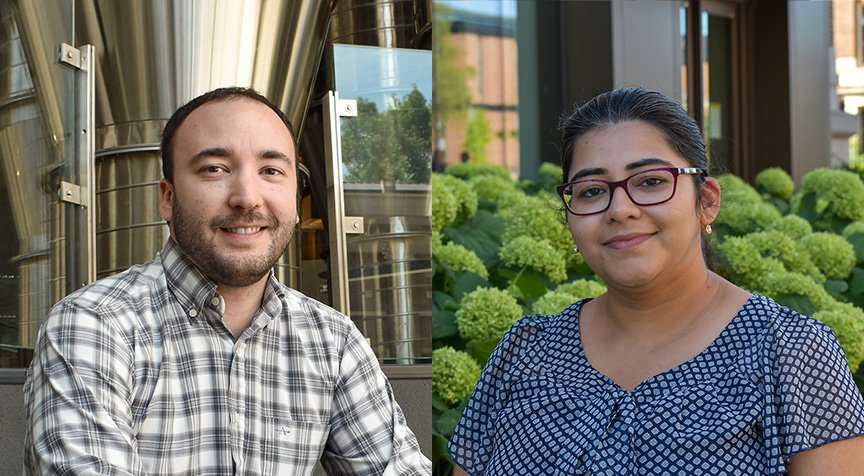
Saumya Sinha
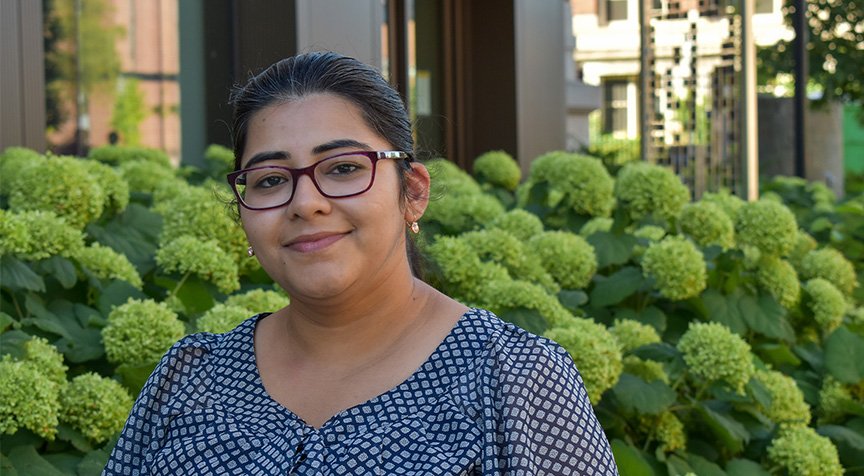
Saumya Sinha brings an impressive background in applied mathematics, solving problems in sequential decision-making, and optimization under uncertainty to the improvement of healthcare and health policy. She joined the ISyE department as an assistant professor in the fall semester of 2022.
Sinha grew up in Delhi, India and has always had a passion for learning. She first got interested in the idea of scientific exploration and research in high school, when she had to study for a math class on her own with limited guidance due to scheduling issues. This turned out to be serendipitous, as she enjoyed the academic freedom to explore and study as she liked, and decided to pursue her interest in math and science.
After getting her bachelor of science from the University of Delhi, she pursued her master’s at the Tata Institute of Fundamental Research Centre for Applicable Mathematics in Bengaluru. She moved to the United States to pursue a Ph.D. in applied mathematics at the University of Washington in Seattle, where she was further exposed to applications of operations research and industrial engineering.
While much of her graduate research was theoretical and highly technical, she sought a broader, application-oriented experience. This drove her to take a subsequent postdoctoral position at Rice University. Her postdoctoral advisor had a long history of healthcare research and she saw an opportunity to apply her mathematics training to study health policy.
Her most recent research focuses on the reaction of transplant hospitals to federal regulations that evaluated hospitals on the basis of patients’ post-transplant outcomes and penalized those with poorer-than-expected outcomes. Sinha’s work demonstrated that these regulations could induce risk-aversion whereby hospitals would turn away sicker but high-risk patients with unpredictable outcomes. She further observed that medium-sized hospitals were disproportionately impacted. While many studies have looked at the problem from the perspective of the patients and the government, not many have analyzed the problem from the hospitals’ perspective, and how organ transplant organizations react to government regulation.
During her study, Sinha sat in on organ transplant medical review board meetings to learn about and analyze how these critical decisions were being made, and how to optimize as many factors as possible to create the best outcome for hospitals and patients. She further learned that while modeling can help these boards make more streamlined and data-driven decisions, it cannot replace subjective conversations regarding who is approved to receive a transplant and who is not. “There are so many variables that cannot be quantified,” she says. “And that is why the intention of this work is always to assist and support the decision-makers, never to replace them. The need [for such tools] is so much greater than what is available.” The people who decide on organ transplant recipients have a great burden to bear, and it’s clear that Sinha cares greatly for their predicament.
This spring, Sinha will be teaching a new elective on healthcare decision-making. She plans to continue her research into organ transplant policies. She is particularly interested in “pay for performance” federal healthcare reimbursement as it relates to organ transplantation. Based on her previous research, she has formulated alternate incentive mechanisms that can help inform policy-makers on how best to help hospitals and their patients.
Martin Zubeldia
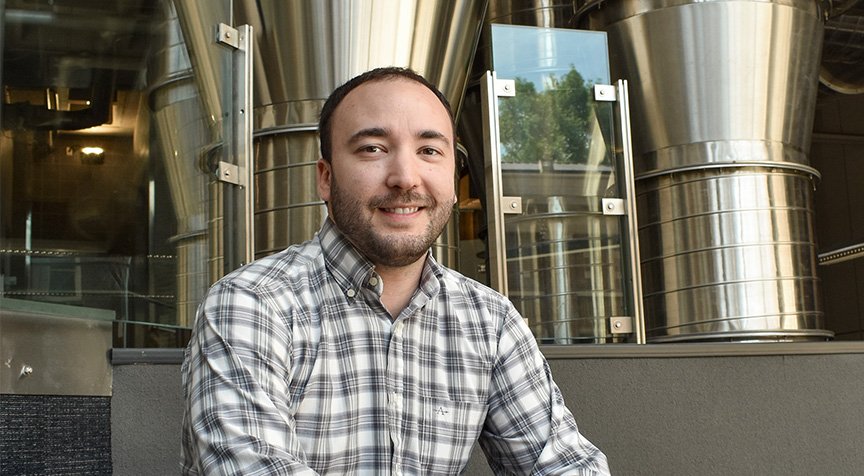
Martín Zubeldía has centered his work around the uncertainties of life. The future is fundamentally unpredictable. Decisions must be made with limited information. But with the power of mathematical modeling, Zubeldía, who joined the ISyE faculty this fall, develops approaches that optimize decision-making in the face of uncertainty and randomness.
Before joining the U of M, Zubeldía held postdoctoral positions for the past three years. Now an ISyE assistant professor, his research has focused on using applied probability for the modeling, analysis, and control of large-scale stochastic decision systems, inspired by applications in computer networks, and other service and learning systems. He is interested in the fundamental trade-offs between performance and efficiency that arise in these systems, with an emphasis on the role that information plays in these tradeoffs.
Zubeldía grew up in Montevideo, Uruguay, the son of an industrial engineer and a high school math teacher. Like many children, he wanted to be an astronaut when he grew up, but his parents encouraged him to try engineering. While he was earning his bachelor’s of science in electronics engineering and a master’s of science in engineering, he discovered that he could turn his love of learning into a career.
His mathematics skills and passion for academics led him into the Massachusetts Institute of Technology, where he completed his Ph.D. in electrical engineering. While in Boston, he experienced snow for the first time. He loved it, but he lamented that the movies didn’t prepare him for how cold snow really is.
From 2019-2021 he was a postdoctoral fellow in the Department of Mathematics and Computer Science at the Eindhoven University of Technology in the Netherlands. Due to the Netherlands’ robust Covid safety policies, much of his experience as a postdoc was remote. While in the Netherlands, he mentored an undergraduate student, and discovered a love for teaching. After a one-year stint as a postdoctoral fellow at the Georgia Institute of Technology, he joined the University of Minnesota as an assistant professor. He is now enjoying living in Minneapolis and experiencing his first Minnesota winter.
In his first semester at the U of M, Zubeldía taught a master’s level course in probability and stochastic models. He is excited about the new and growing department and the possibilities for collaboration with graduate students and further research in his field. He’s hoping to expand his work into machine learning. “Computer scientists are good at creating new machine learning algorithms and testing their limits empirically. I am interested in proving theorems that provide security guarantees for those algorithms.” For example, it’s not very well known how likely or how often reinforcement learning algorithms—such as those in self-driving cars—might make mistakes given a certain number of training hours. He hopes to develop a mathematical framework that can estimate that likelihood. “When you think abstractly about a practical problem, and prove theorems about the abstraction, you discover that the same insights that you obtained can be applied to many different problems.”