Healing the body using machine learning
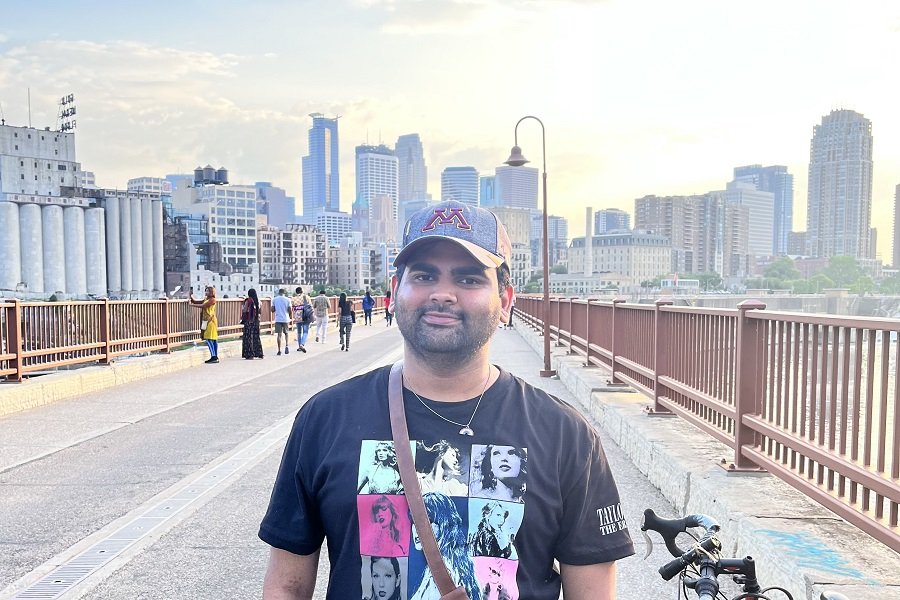
Sai Sanjay Balaji shares his research on biomedical applications of machine learning and what drives his interest in the area
Doctoral candidate and recipient of the 2023 UMII-MnDRIVE Graduate Assistantship Sai Sanjay Balaji is conducting research at the intersection of engineering and medicine. Working under the guidance of Professor Keshab Parhi (Erwin A. Kelen Chair in Electrical Engineering), Sanjay is exploring applications of machine learning for diagnostic purposes. Located at the cutting edge of advancements in healthcare, his research holds the potential to change the way diseases are diagnosed and treatment options are evaluated for a range of illnesses.
Before starting in the graduate program in ECE, Sanjay earned his undergraduate degree in electronics and instrumentation engineering from Anna University, Chennai in India. Here in his words, Sanjay shares his interests, goals, and motivation for pursuing brain research.
What motivated you to come to the U?
I have always been keen on working in the healthcare sector. When I applied to the graduate program here, my primary goal was to enhance my knowledge and skills in the area. The University’s location is ideal. The Twin Cities offers a vibrant ecosystem of healthcare-related businesses, startups, and institutions that present numerous career and networking opportunities. Besides, we have an exceptional medical school, are in close proximity to major healthcare institutions such as the Mayo Clinic, and have a dedicated interdisciplinary research organization, IEM. These resources can broaden my exposure to the medical field and provide collaborative opportunities to understand clinical perspectives better. Of course, I can't overlook the Minnesota winter! Hailing from the southern part of India, where the climate is predominantly warm and humid throughout the year, experiencing a completely different weather is exciting for me. I absolutely adore the winter!
What are your research interests?
My interest lies in the application of machine learning (ML) on biosignals. Specifically, I would like to use machine learning, deep learning, and other artifical intelligence approaches to make accurate and efficient diagnoses as they have the potential to speed up the process, increase accuracy, and improve patient outcomes. I would also like apply ML techniques to analyze biosignal data (that serve as markers of specific diseases), identify informative features, and develop models for biomarker detection. Lastly, I am also interested in integrating ML algorithms into a feedback loop that continuously monitors a patient's physiological signals or health status and delivers personalized interventions or treatments in real time. This would allow for an automated and more importantly, adaptive approach to patient care.
What are the highlights of your dissertation-specific research?
My dissertation research is focused on understanding brain interactions through a graph representation and using these to create algorithms for diagnosing medical conditions, including neurological disorders, brain-related diseases, and cognitive assessments.
The recent advancements in graph-based deep learning models offer a promising framework for representing brain networks and developing subsequent algorithms. My first set of research projects focused on classifying patients with prefrontal cortex (pFC) lesions from healthy controls using a graph neural network model. In our study, we found that even a simplified graph model of the brain with only 20 percent of its connections can effectively tell the difference between two groups of people. We also provide insights into how important different parts of the brain are in processing working memory.
Supported by the UMII-MnDRIVE assistantship, I am currently developing an algorithm for locating the seizure onset zone (SOZ) from intracranial electroencephalogram (EEG) signals. The goal is to build a robust graph-based model that can automate the process of seizure localization, which clinicians currently do manually by observing hours of electrophysiological data, a cumbersome process that is occasionally prone to errors.
What is your motivation for pursuing brain-related research?
My fascination with the brain is what draws me to this field. As the control center of our bodies, the brain orchestrates an array of functions, from cognitive processes like thinking and learning to the regulation of essential bodily functions such as breathing and heartbeat. Its remarkable swiftness and computational power to rapidly process and respond to sensory stimuli match a supercomputer's ability. The brain's adaptability is another point of fascination; it can reconfigure its structure by forming new connections between neurons in response to new experiences. Astonishingly enough, this intricate network of billions of neurons manages to accomplish all these tasks while consuming just between 12 to 20 watts of power, a feat that appears impossible to replicate in constructing an equivalent computer. On an aside, isn’t it amusing how I'm employing 'artificial' neural networks to unravel the mysteries of 'real' neural networks?
What are some key impacts you see stemming from your overall research?
Causal brain network analysis can offer a deeper understanding of brain function by unveiling how different regions causally interact, shedding light on the core aspects of brain operation. This knowledge can facilitate early diagnosis and targeted treatments for neurological and psychiatric disorders, aid in rehabilitation strategies, and enhance brain-computer interfaces for individuals with disabilities. Additionally, personalized medicine could benefit from insights into individual brain network variations, enabling tailored treatments. Research in the broad area can contribute to advancements in neuroscience, fuel technological innovations, and foster interdisciplinary collaboration. In essence, this research area can transform our understanding of the brain, and lead to improved healthcare through better diagnosis, treatments, and treatment technologies.
Where do you hope to apply your expertise eventually?
As a Ph.D. student working at the intersection of neuroscience and machine learning, my short to mid-term goal is to apply my expertise to connect the realms of academic research and industry applications. I hope to gain industry experience to understand the feasibility and practicality of various machine learning algorithms in the context of neuroscience tasks such as closed-loop treatment of epilepsy, continuous monitoring of Parkinson's disease, predicting depression and anxiety, detecting strokes, diagnosing Alzheimer's disease, enabling brain-computer interfaces for paralysis, seizure prediction, psychiatric disorder diagnosis, traumatic brain injury outcome prediction, and personalized treatment plans.
Ultimately however, I would like to return to academia and conduct research that bridges the gap between theoretical knowledge and practical applications in neuroscience and machine learning.
Earlier this year, Sai Sanjay Balaji’s paper was recognized with the Best Student Paper award at the 11th International IEEE EMBS Conference on Neural Engineering. The paper was titled, “Classifying subjects with PFC lesions from healthy controls during working memory encoding via graph convolutional networks.”