Chemistry for computing
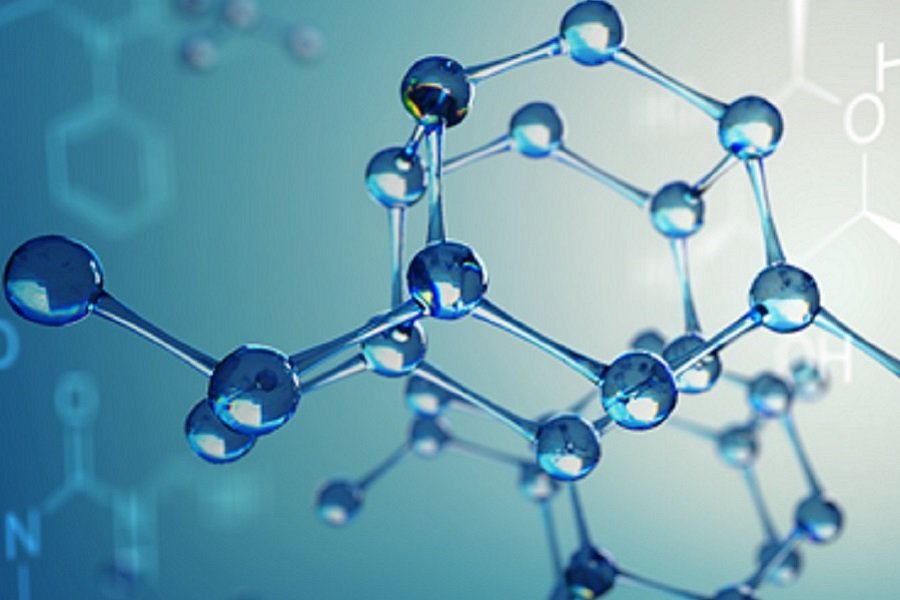
Scientists demonstrate use of acid-base reactions for information storage and processing
In a collaborative endeavor, ECE’s Professor Marc Riedel and scientists from Brown University have demonstrated the use of acid-base reactions to encode binary information and perform information processing operations to build digital circuits and neural networks. The details of their study are published in Nature Communications in a paper titled “Digital circuits and neural networks based on acid-base chemistry implemented by robotic fluid handling.” Their work is particularly significant as it demonstrates an alternative to conventional semiconductor-based technologies for data processing and information storage.
Commenting on the significance of the team's research, Riedel says: Over the past two decades, computing has moved from desktops and data centers into the wild. Embedded microchips in our gadgets, our tools, and even our bodies are transforming our lives. And yet, there are limits to where silicon can compute effectively. It requires a power source and even miniaturized to the microscale or smaller, an electronic system is a foreign object inserted into a material or environment. Imagine if we could build devices that compute directly in their chemical environment such as our bodies?
Research into alternatives to semiconductor devices is imperative for reasons such as increased power efficiency, affordability, and adaptability. Molecular computing is a particularly promising computing paradigm as its reliance on chemical reactions makes it potentially more space and energy efficient. In the present study, the team has proposed the use of acids, bases, and their reactions to store and process information based on majority and negation operations. The two operations can be used to carry out the complex calculations that define digital circuits and neural networks. In the paper the scientists lay the groundwork by providing the building blocks for setting up digital circuits using acids and bases, and demonstrate the use of acid-base reactions to implement a neural network classifier.
The chemical approach proposed by the team is direct; it involves the mixing of a strong acid with a strong base. The mixing executes the majority function, and the inverse function is brought about by reversing the roles of the complementary pair (acid-base pair or base-acid pair). Both majority and inversion operations are complete functions which means they can be used to build logic gates (such as AND, NAND, OR, NOR, etc.) to perform any logic function.
Following the successful presentation of logic gates derived from chemical reactions, the team demonstrated the use of acid-base reactions for classification, a task commonly undertaken by neural networks. Neural networks typically use available datasets to derive relationships to help them classify. This learning is done in the training phase, which is followed by the inference phase where the training is applied on new information outside the dataset. In the present study, the team deployed the chemical reaction approach in the inference phase. The outcome indicated a high level of accuracy (approximately 99 percent) which indicates that the chemical approach could potentially be used for the design of neural networks.
A notable limitation of using acid-base reactions for the depiction of logic functions is that the pH moves progressively closer to neutral as the reaction progresses across multiple gates. The scientists have suggested two possible ways to address the issue. One possibility is the use of pH-sensitive valves that can restore pH-levels so that the computation can be sustained over a longer chain of reactions. Another approach would be to implement digital logic optimization algorithms to reduce the number of logic layers. This will cut down the number of reactions thereby minimizing the dilution of the reactants.
Riedel views the research as a game changer for the information technology industry: "Computing with chemistry presents a largely unexplored challenge: constructing programmable, composable molecular computing systems from the bottom up, starting with constituent reactions that are not inherently programmable. This research is the first notable success for this sort of chemical computation. It will open the door for research into many other forms, particularly with small molecules (as compared to large ones such as DNA and proteins)."
The path ahead
Various molecular computing methodologies have been proposed and explored by the scientific community of which DNA computing has become the most widely studied form. It relies on DNA strands as information carriers that will execute mathematical and logical operations. But the method is not without its challenges: temperature sensitivities, long computation times, dependence on specific and large numbers of reagents are just some of the limitations that have acted as constraints to its widespread use. In such a scenario, the successful demonstration of acid-base chemistry to conduct computations points to the possibility of avoiding the more complex spatio-temporal challenges that define some of the other unconventional computation methods previously explored. Of particular significance is that the current approach offers the advantage of speed given how quickly a strong acid can react with a strong base giving further strength to the possibility of using the approach for data storage and processing.
Looking forward, the study authors are considering the use of weak acids and bases as pH buffers, which could open up the possibility of using the chemical approach for more complex computations which are critical for multilayer neural networks.
Read the article, "Digital circuits and neural networks based on acid-base chemistry implemented by robotic fluid handling" in Nature Communications