Clustering augmented Self-Supervised Learning: An application to Land Cover Mapping [conference paper]
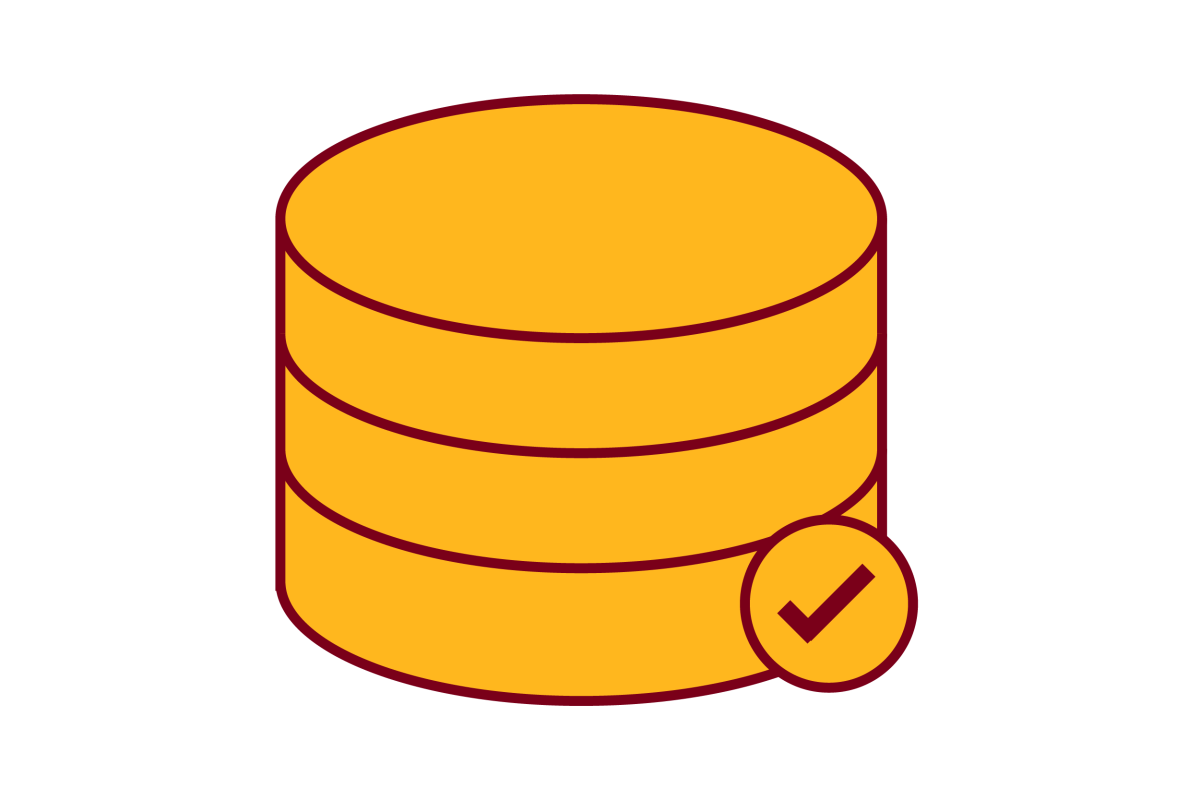
Conference
2nd ACM SIGKDD Workshop on Deep Learning for Spatiotemporal Data, Applications, and Systems (DEEPSPATIAL 2021) - August 15, 2021
Authors
Rahul Ghosh (Ph.D. student), Xiaowei Jia (Ph.D. 2020), Chenxi Lin, Zhenong Jin, Vipin Kumar (professor)
Abstract
Collecting large annotated datasets in Remote Sensing is often expensive and thus can become a major obstacle for training advanced machine learning models. Common techniques of addressing this issue, based on the underlying idea of pre-training the Deep Neural Networks (DNN) on freely available large datasets, cannot be used for Remote Sensing due to the unavailability of such large-scale labeled datasets and the heterogeneity of data sources caused by the varying spatial and spectral resolution of different sensors. Selfsupervised learning is an alternative approach that learns feature representation from unlabeled images without using any human annotations. In this paper, we introduce a new method for land cover mapping by using a clustering based pretext task for self-supervised learning. We demonstrate the effectiveness of the method on two societally relevant applications from the aspect of segmentation performance, discriminative feature representation learning and the underlying cluster structure. We also show the effectiveness of the active sampling using the clusters obtained from our method in improving the mapping accuracy given a limited budget of annotating.
Link to full paper
Clustering augmented Self-Supervised Learning: An application to Land Cover Mapping
Keywords
spatial computing, deep learning