FERN: Fair Team Formation for Mutually Beneficial Collaborative Learning [preprint]
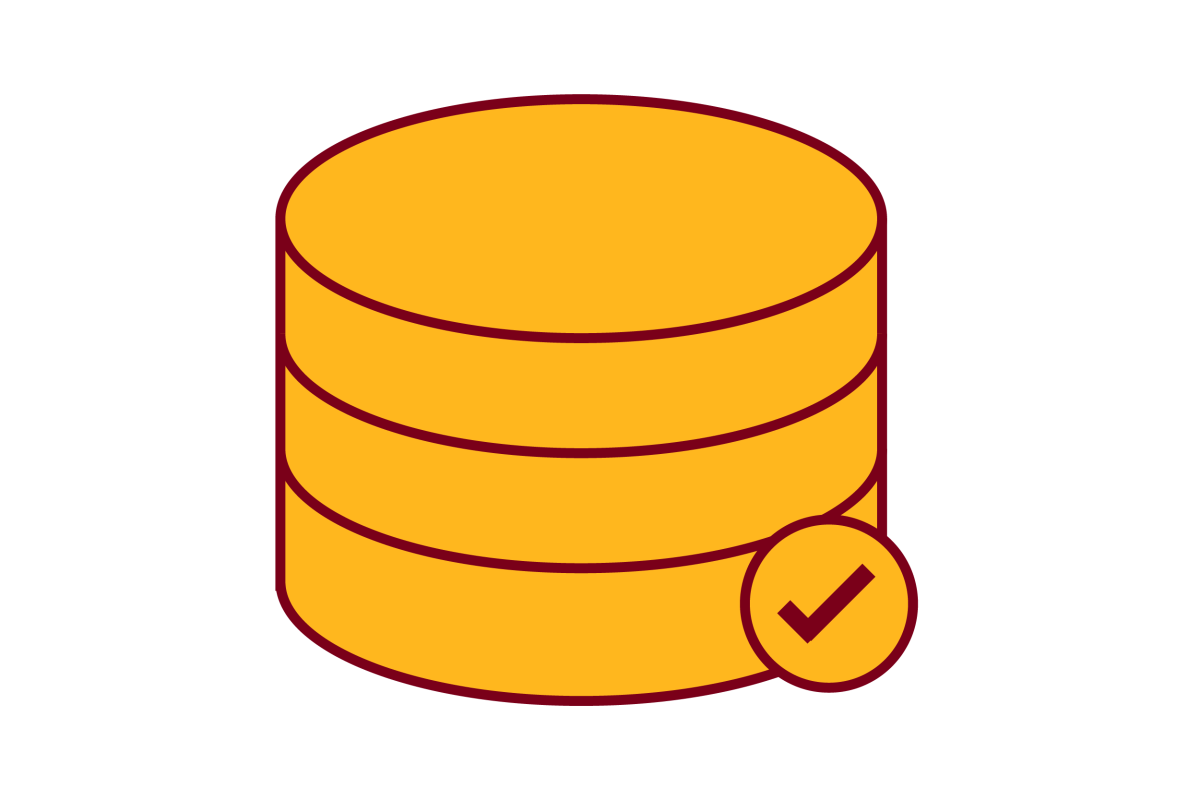
Preprint date
November 23, 2020
Authors
Maria Kalantzi (Ph.D. student), Agoritsa Polyzou (Ph.D. 2020), George Karypis (professor)
Abstract
Automated Team Formation is becoming increasingly important for a plethora of applications in open source community projects, remote working platforms, as well as online educational systems. The latter case, in particular, poses significant challenges that are specific to the educational domain. Indeed, teaming students aims to accomplish far more than the successful completion of a specific task. It needs to ensure that all members in the team benefit from the collaborative work, while also ensuring that the participants are not discriminated with respect to their protected attributes, such as race and gender. Towards achieving these goals, this work introduces FERN, a fair team formation approach that promotes mutually beneficial peer learning, dictated by protected group fairness as equality of opportunity in collaborative learning. We formulate the problem as a multi-objective discrete optimization problem. We show this problem to be NP-hard and propose a heuristic hill-climbing algorithm. Extensive experiments on both synthetic and real-world datasets against well-known team formation techniques show the effectiveness of the proposed method.
Link to full paper
FERN: Fair Team Formation for Mutually Beneficial Collaborative Learning
Keywords
fairness, recommender systems, data mining