Surface Normal Estimation of Tilted Images via Spatial Rectifier [conference paper]
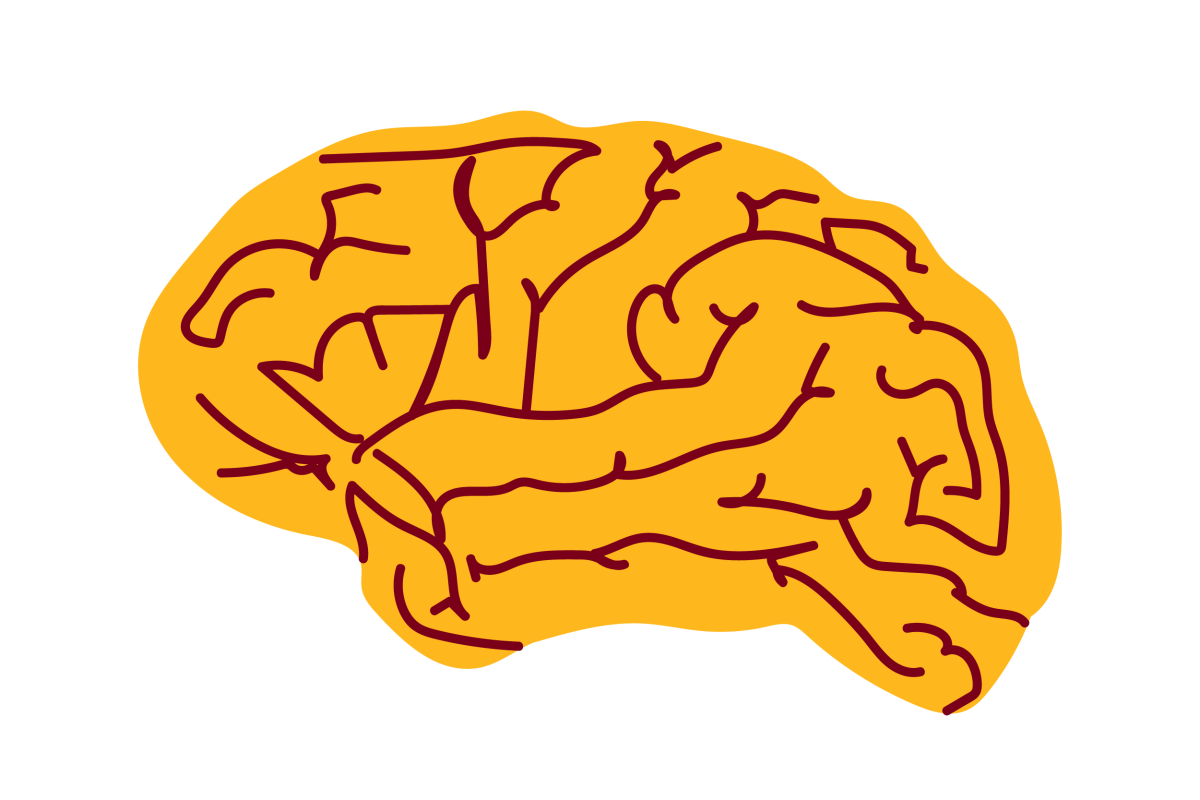
Conference
European Conference on Computer Vision - August 23, 2020
Authors
Tien Do (Ph.D. student), Khiem Vuong (undergraduate research assistant), Stergios I. Roumeliotis (adjunct professor), Hyun Soo Park (assistant professor)
Abstract
In this paper, we present a spatial rectifier to estimate surface normals of tilted images. Tilted images are of particular interest as more visual data are captured by arbitrarily oriented sensors such as body-/robot-mounted cameras. Existing approaches exhibit bounded performance on predicting surface normals because they were trained using gravity-aligned images. Our two main hypotheses are: (1) visual scene layout is indicative of the gravity direction; and (2) not all surfaces are equally represented by a learned estimator due to the structured distribution of the training data, thus, there exists a transformation for each tilted image that is more responsive to the learned estimator than others. We design a spatial rectifier that is learned to transform the surface normal distribution of a tilted image to the rectified one that matches the gravity-aligned training data distribution. Along with the spatial rectifier, we propose a novel truncated angular loss that offers a stronger gradient at smaller angular errors and robustness to outliers. The resulting estimator outperforms the state-of-the-art methods including data augmentation baselines not only on ScanNet and NYUv2 but also on a new dataset called Tilt-RGBD that includes considerable roll and pitch camera motion.
Link to project website
Surface Normal Estimation of Tilted Images via Spatial Rectifier
Keywords
computer vision