TrashCan: A Semantically-Segmented Dataset towards Visual Detection of Marine Debris [preprint]
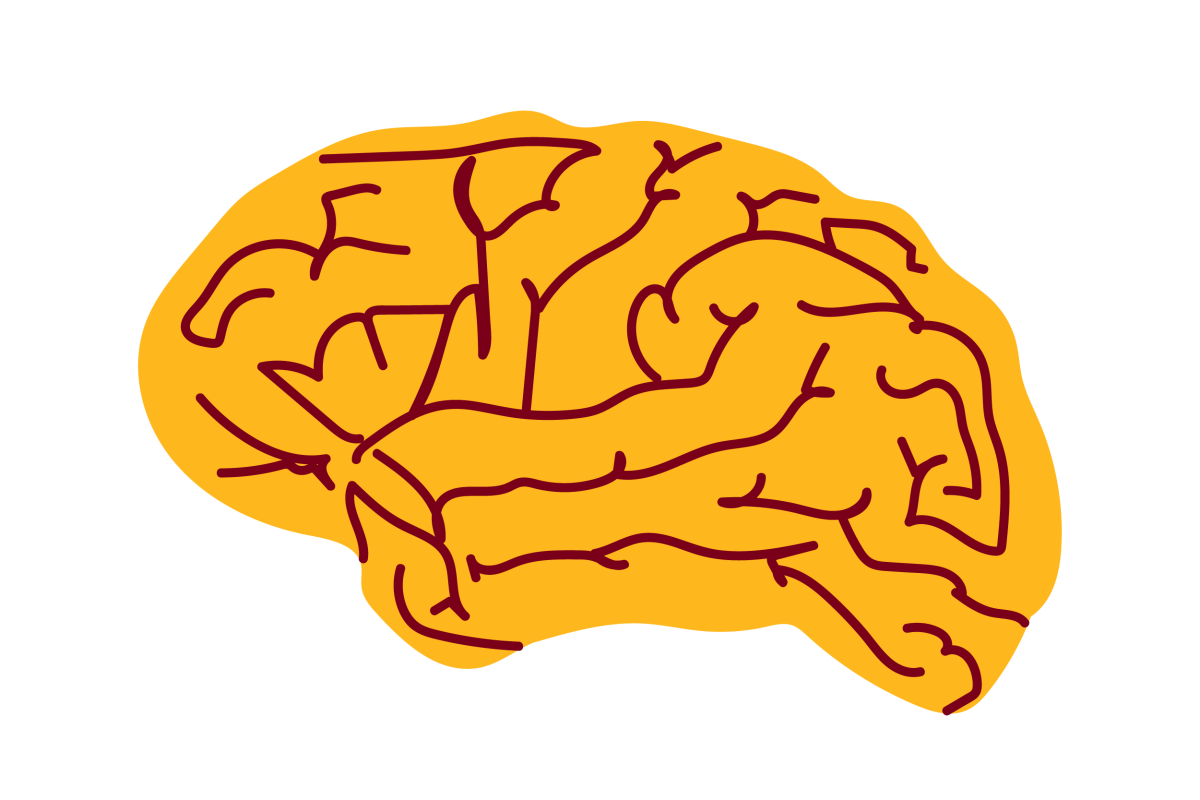
Preprint date
July 16, 2020
Authors
Jungseok Hong (Ph.D. student), Michael Fulton (Ph.D. student), Junaed Sattar (assistant professor)
Abstract
This paper presents TrashCan, a large dataset comprised of images of underwater trash collected from a variety of sources, annotated both using bounding boxes and segmentation labels, for development of robust detectors of marine debris. The dataset has two versions, TrashCan-Material and TrashCan-Instance, corresponding to different object class configurations. The eventual goal is to develop efficient and accurate trash detection methods suitable for onboard robot deployment. Along with information about the construction and sourcing of the TrashCan dataset, we present initial results of instance segmentation from Mask R-CNN and object detection from Faster R-CNN. These do not represent the best possible detection results but provides an initial baseline for future work in instance segmentation and object detection on the TrashCan dataset.
Link to full paper
TrashCan: A Semantically-Segmented Dataset towards Visual Detection of Marine Debris
Keywords
computer vision, robotics