3D model processing for high throughput phenotype extraction–the case of corn [journal]
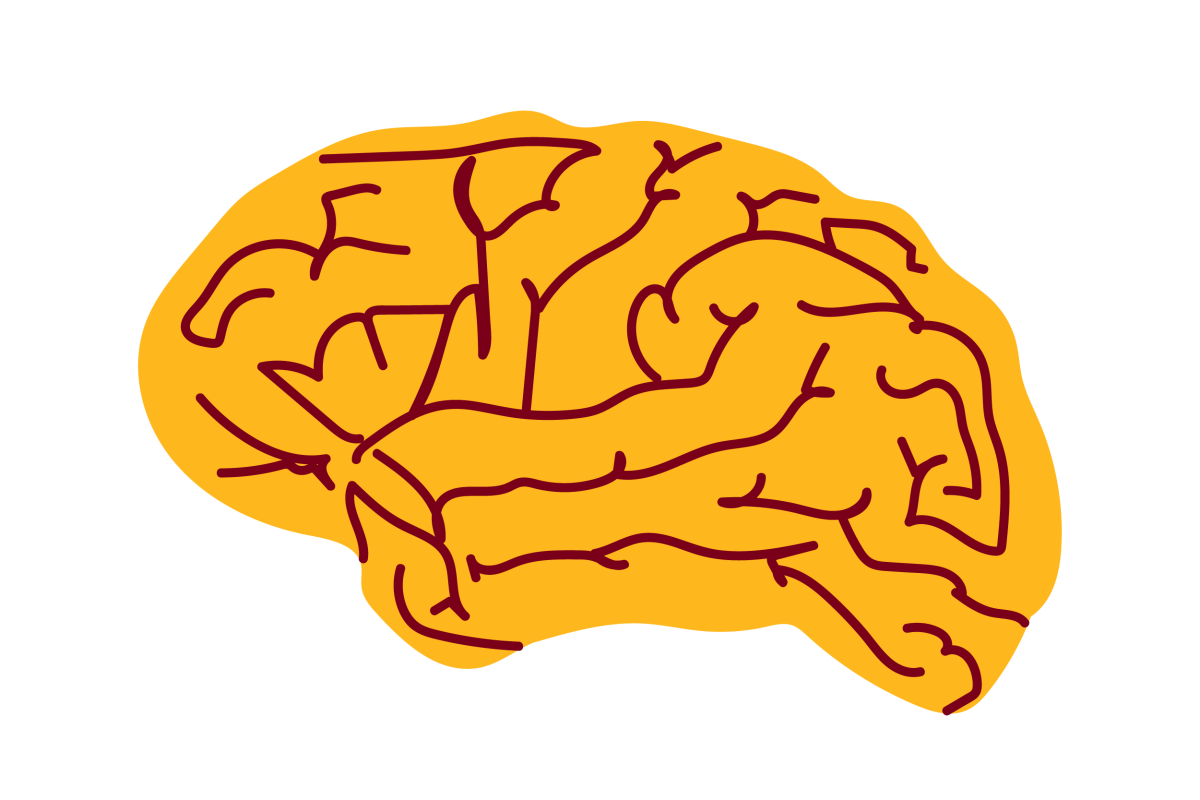
Journal
Computers and Electronics in Agriculture - May 1, 2020
Authors
Dimitris Zermas (Ph.D. 2018), Vassilios Morellas (research director), David Mulla, Nikos Papanikolopoulos (professor)
Abstract
High resolution RGB imagery collected using a UAV and a handheld camera was used with structure from motion to reconstruct 3D canopies of small groups of corn plants. A methodology for the automated extraction of phenotypic characteristics of individual plants is presented based on these 3D reconstructed canopies. Such information can enhance the evaluation of crop traits and provide accurate and frequent statistics for in-season assessment of their changes with growth stage. Industries that target yield optimization and crop hybrid production can benefit greatly from this approach. The use of 3D models provides elevated information content, when compared to alternative planar methods, mainly due to the alleviation of leaf occlusions. High resolution images of corn stalks are collected and used to obtain 3D models for individual plants. Based on those extracted 3D point clouds, the calculation of phenotypic characteristics are obtained, such as the number of plants in an area, the Leaf Area Index (LAI), the individual and average plant height, the individual leaf length, the location and the angles of leaves with respect to the stem. An experimental validation using both artificial corn plants emulating real world scenarios and real corn plants in different growth stages, supports the accuracy of the proposed methodology.
Link to full paper
3D model processing for high throughput phenotype extraction–the case of corn
Keywords
robotics, agriculture