Attention-augmented Spatio-Temporal Segmentation for Land Cover Mapping [preprint]
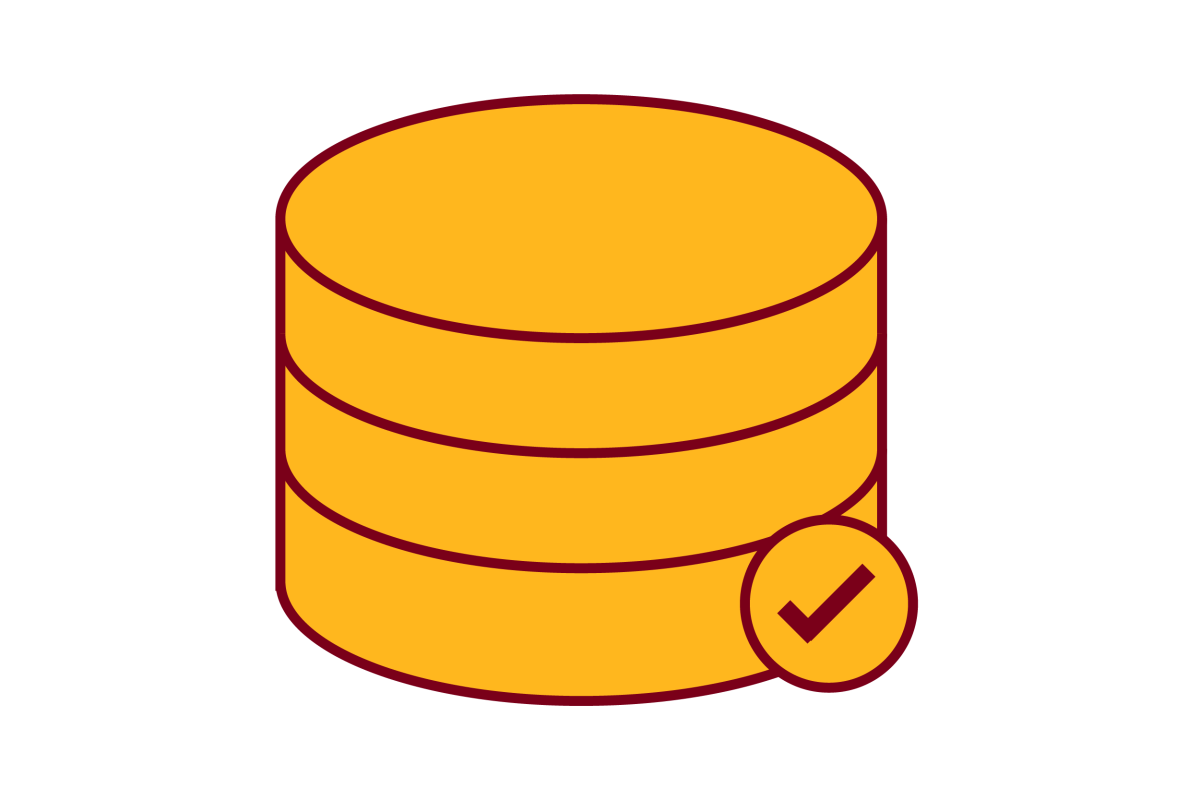
Preprint date
May 2, 2021
Authors
Rahul Ghosh (Ph.D. student), Praveen Ravirathinam, Xiaowei Jia (Ph.D. 2020), Chenxi Lin, Zhenong Jin, Vipin Kumar (professor)
Abstract
The availability of massive earth observing satellite data provide huge opportunities for land use and land cover mapping. However, such mapping effort is challenging due to the existence of various land cover classes, noisy data, and the lack of proper labels. Also, each land cover class typically has its own unique temporal pattern and can be identified only during certain periods. In this article, we introduce a novel architecture that incorporates the UNet structure with Bidirectional LSTM and Attention mechanism to jointly exploit the spatial and temporal nature of satellite data and to better identify the unique temporal patterns of each land cover. We evaluate this method for mapping crops in multiple regions over the world. We compare our method with other state-of-the-art methods both quantitatively and qualitatively on two real-world datasets which involve multiple land cover classes. We also visualize the attention weights to study its effectiveness in mitigating noise and identifying discriminative time period.
Link to full paper
Attention-augmented Spatio-Temporal Segmentation for Land Cover Mapping
Keywords
spatial computing, data mining