CalCROP21: A Georeferenced multi-spectral dataset of Satellite Imagery and Crop Labels [preprint]
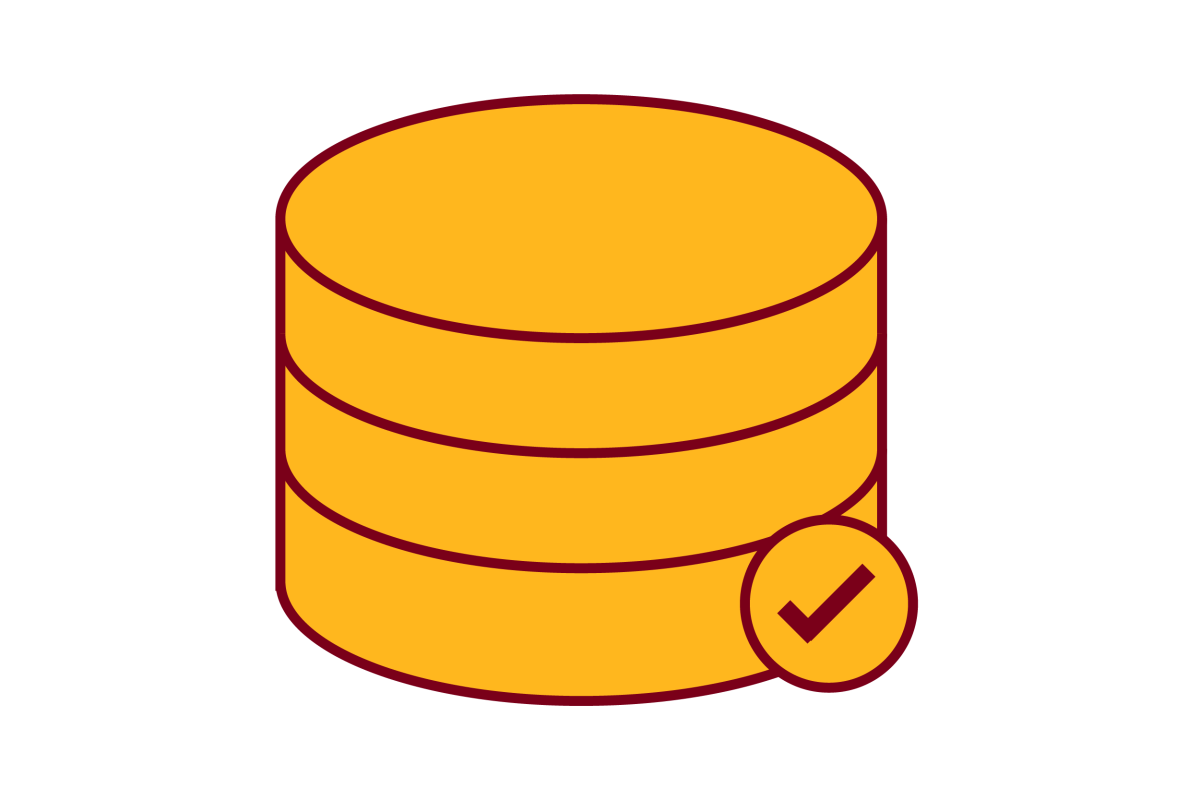
Preprint date
July 26, 2021
Authors
Rahul Ghosh (Ph.D. student), Praveen Ravirathinam (research assistant), Xiaowei Jia (Ph.D. 2020), Ankush Khandelwal (Ph.D. 2019), David Mulla, Vipin Kumar (professor)
Abstract
Mapping and monitoring crops is a key step towards sustainable intensification of agriculture and addressing global food security. A dataset like ImageNet that revolutionized computer vision applications can accelerate development of novel crop mapping techniques. Currently, the United States Department of Agriculture (USDA) annually releases the Cropland Data Layer (CDL) which contains crop labels at 30m resolution for the entire United States of America. While CDL is state of the art and is widely used for a number of agricultural applications, it has a number of limitations (e.g., pixelated errors, labels carried over from previous errors and absence of input imagery along with class labels). In this work, we create a new semantic segmentation benchmark dataset, which we call CalCROP21, for the diverse crops in the Central Valley region of California at 10m spatial resolution using a Google Earth Engine based robust image processing pipeline and a novel attention based spatio-temporal semantic segmentation algorithm STATT. STATT uses re-sampled (interpolated) CDL labels for training, but is able to generate a better prediction than CDL by leveraging spatial and temporal patterns in Sentinel2 multi-spectral image series to effectively capture phenologic differences amongst crops and uses attention to reduce the impact of clouds and other atmospheric disturbances. We also present a comprehensive evaluation to show that STATT has significantly better results when compared to the resampled CDL labels. We have released the dataset and the processing pipeline code for generating the benchmark dataset.
Link to full paper
CalCROP21: A Georeferenced multi-spectral dataset of Satellite Imagery and Crop Labels
Keywords
spatial computing, environmental data science