Developing a Recommendation Benchmark for MLPerf Training and Inference [preprint]
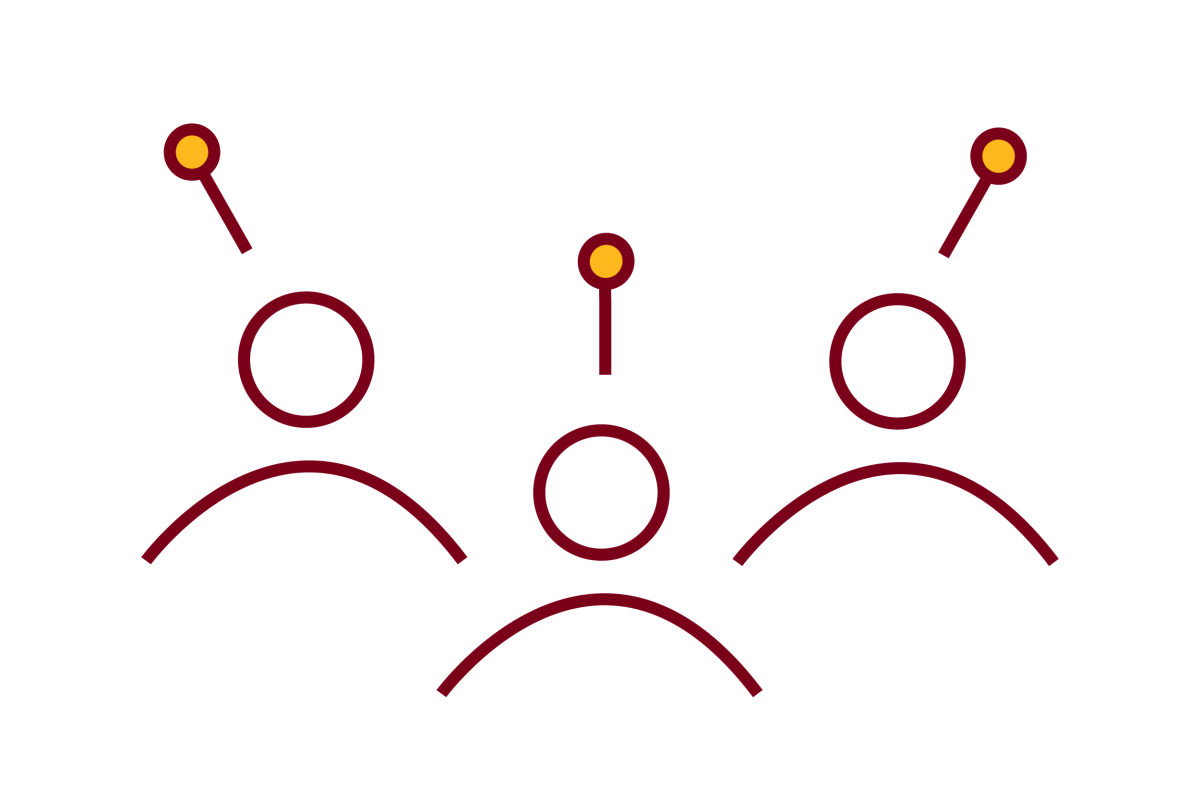
Preprint date
April 14, 2020
Authors
Carole-Jean Wu, Robin Burke, Ed H. Chi, Joseph Konstan (professor), Julian McAuley, Yves Raimond, Hao Zhang
Abstract
Deep learning-based recommendation models are used pervasively and broadly, for example, to recommend movies, products, or other information most relevant to users, in order to enhance the user experience. Among various application domains which have received significant industry and academia research attention, such as image classification, object detection, language and speech translation, the performance of deep learning-based recommendation models is less well explored, even though recommendation tasks unarguably represent significant AI inference cycles at large-scale datacenter fleets. To advance the state of understanding and enable machine learning system development and optimization for the commerce domain, we aim to define an industry-relevant recommendation benchmark for the MLPerf Training andInference Suites. The paper synthesizes the desirable modeling strategies for personalized recommendation systems. We lay out desirable characteristics of recommendation model architectures and data sets. We then summarize the discussions and advice from the MLPerf Recommendation Advisory Board.
Link to full paper
Developing a Recommendation Benchmark for MLPerf Training and Inference
Keywords
recommender systems, human computer interaction (HCI), social computing