GraphInf: A GCN-based Popularity Prediction System for Short Video Networks [conference app
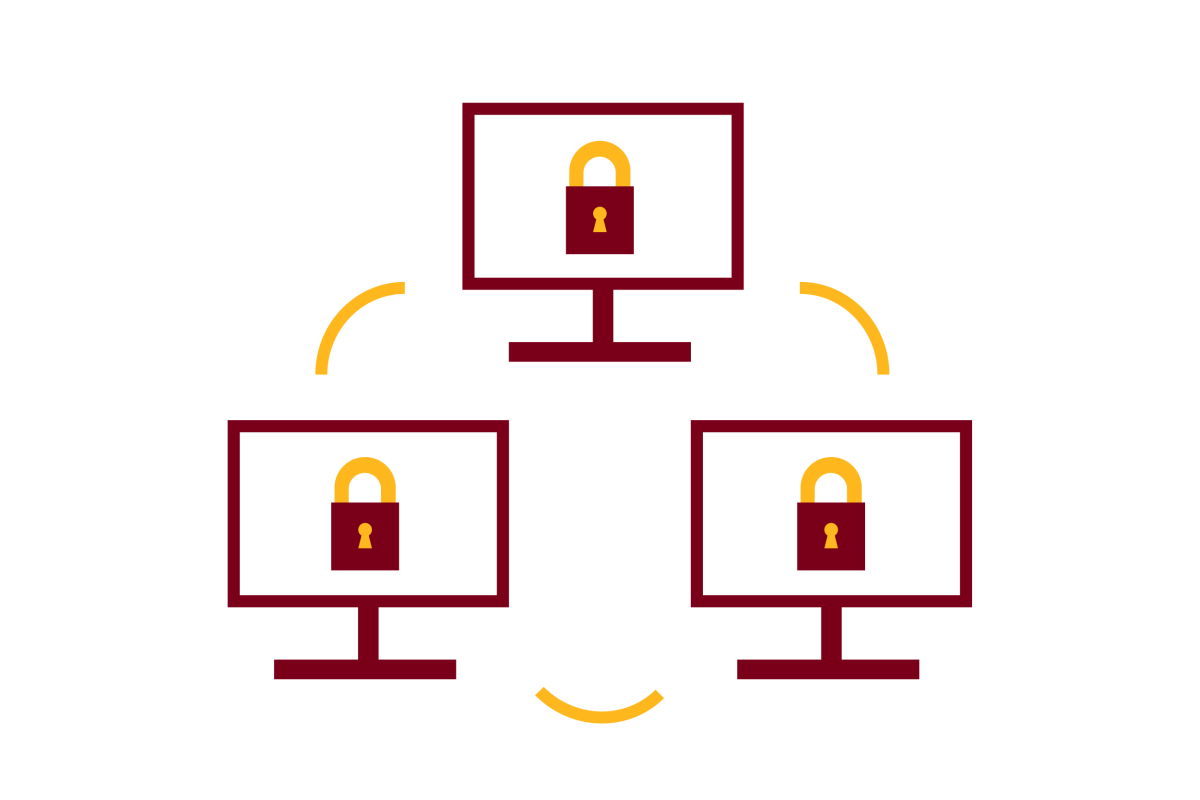
Conference
27th International Conference on Web Services (ICWS) - September 18-20, 2020
Authors
Yuchao Zhang, Pengmiao Li, Zhi-Li Zhang (professor), Chaorui Zhang, Wendong Wang, Yishuang Ning, Bo Lian
Abstract
As the emerging entertainment applications, short video platforms, such as Youtube, Kuaishou, quickly dominant the Internet multimedia traffic. The caching problem will surely provide a great reference to network management (e.g., traffic engineering, content delivery). The key to cache is to make precise popularity prediction. However, different from traditional multimedia applications, short video network exposes unique characteristics on popularity prediction due to the explosive video quantity and the mutual impact among these countless videos, making the state-of-the-art solutions invalid. In this paper, we first give an in-depth analysis on 105,231,883 real traces of 12,089,887 videos from Kuaishou Company, to disclose the characteristics of short video network. We then propose a graph convolutional neural-based video popularity prediction algorithm called GraphInf. In particular, GraphInf clusters the countless short videos by region and formulates the problem in a graph-based way, thus addressing the explosive quantity problem. GraphInf further models the influence among these regions with a customized graph convolutional neural (GCN) network, to capture video impact. Experimental results show that GraphInf outperforms the traditional Graph-based methods by 44.7%. We believe such GCN-based popularity prediction would give a strong reference to related areas.
Link to full paper
GraphInf: A GCN-based Popularity Prediction System for Short Video Networks
Keywords
networking