Lumos5G: Mapping and Predicting Commercial mmWave 5G Throughput [conference paper]
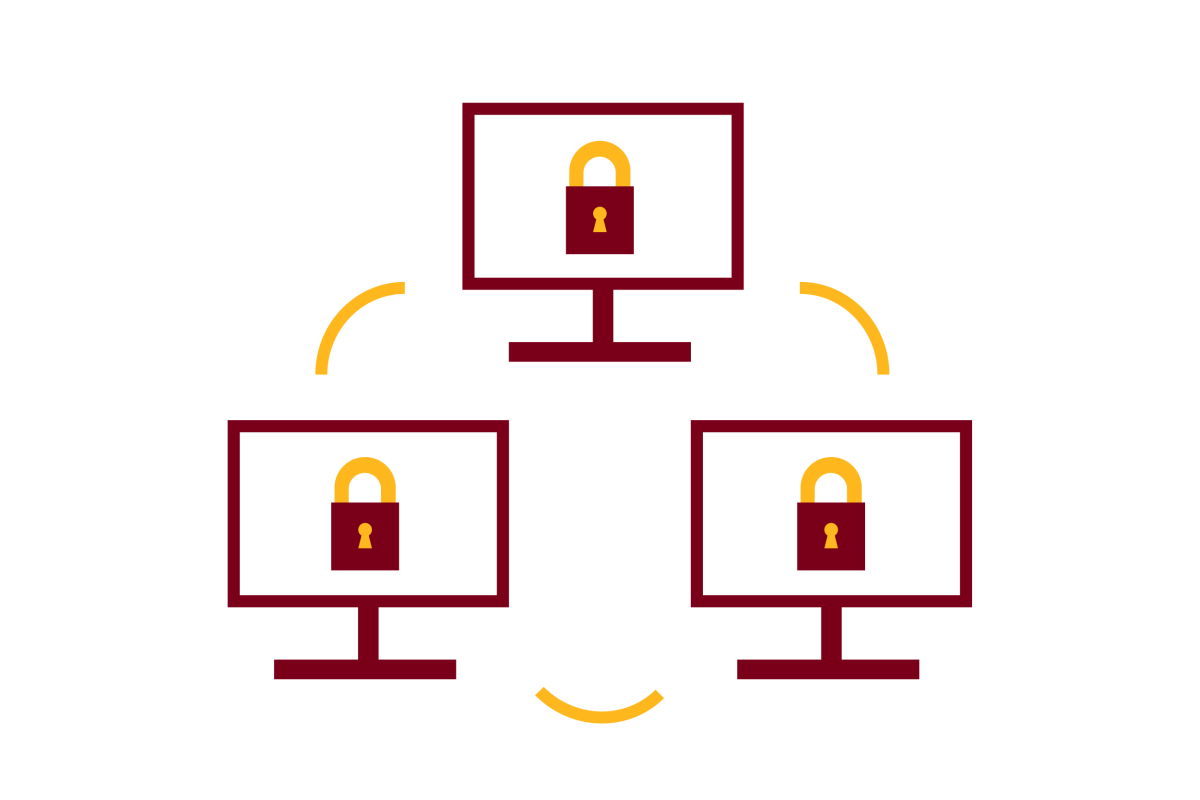
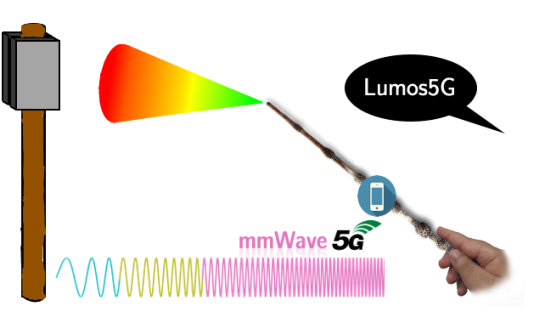
Conference
Proceedings of the ACM Internet Measurement Conference - October 27, 2020
Authors
Arvind Narayanan (Ph.D. student), Eman Ramadan (Ph.D. student), Rishabh Mehta (M.S. student), Xinyue Hu (Ph.D. student), Qingxu Liu (undergraduate research assistant), Rostand AK Fezeu (Ph.D. student), Udhaya Kumar Dayalan (Ph.D. student), Saurabh Verma (Ph.D. student), Peiqi Ji (undergraduate research assistant), Tao Li, Feng Qian (assistant professor), Zhi-Li Zhang (professor)
Abstract
The emerging 5G services offer numerous new opportunities for networked applications. In this study, we seek to answer two key questions: i) is the throughput of mmWave 5G predictable, and ii) can we build "good" machine learning models for 5G throughput prediction? To this end, we conduct a measurement study of commercial mmWave 5G services in a major U.S. city, focusing on the throughput as perceived by applications running on user equipment (UE). Through extensive experiments and statistical analysis, we identify key UE-side factors that affect 5G performance and quantify to what extent the 5G throughput can be predicted. We then propose Lumos5G - a composable machine learning (ML) framework that judiciously considers features and their combinations, and apply state-of-the-art ML techniques for making context-aware 5G throughput predictions. We demonstrate that our framework is able to achieve 1.37x to 4.84x reduction in prediction error compared to existing models. Our work can be viewed as a feasibility study for building what we envisage as a dynamic 5G throughput map (akin to Google traffic map). We believe this approach provides opportunities and challenges in building future 5G-aware apps.
Link to full paper
Lumos5G: Mapping and Predicting Commercial mmWave 5G Throughput
Keywords
mobile networks, networking