A Methodology for the Detection of Nitrogen Deficiency in Corn Fields Using High-Resolution RGB Imagery [journal]
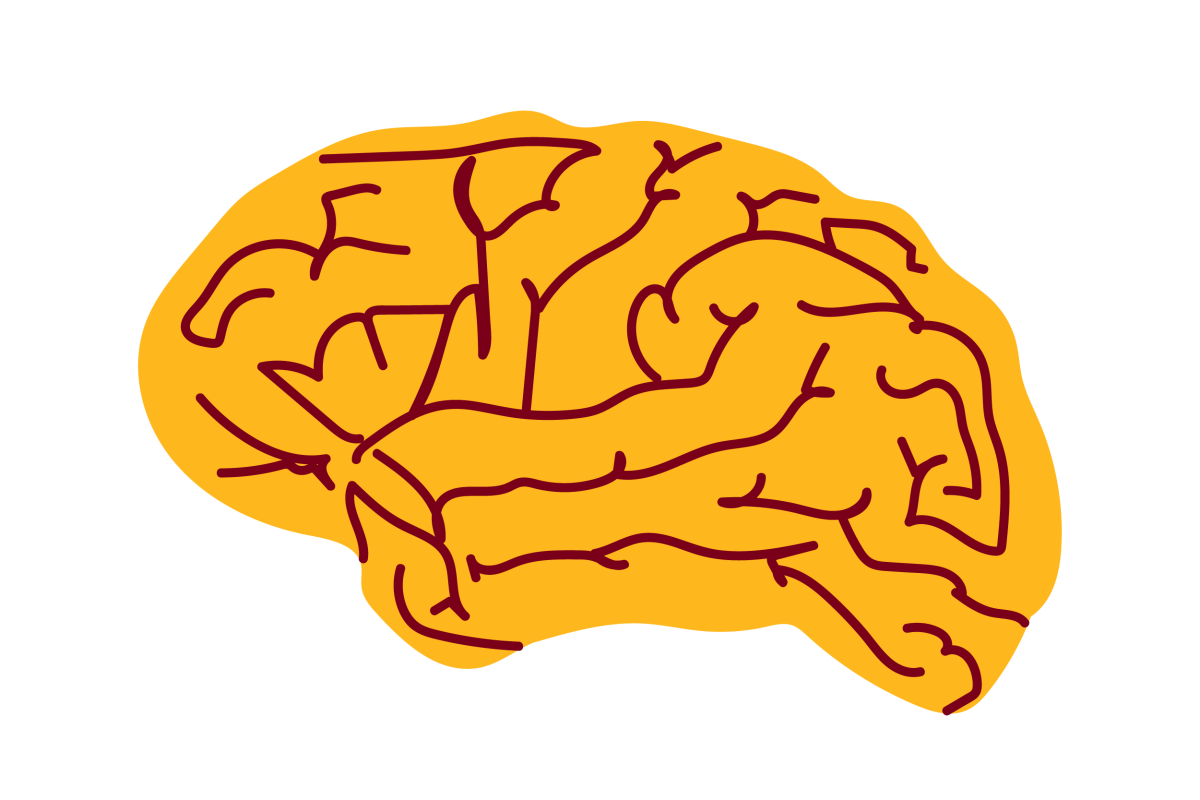
Journal
IEEE Transactions on Automation Science and Engineering - October 7, 2020
Authors
Dimitris Zermas, Henry J Nelson (Ph.D. student), Panagiotis Stanitsas (Ph.D. 2018), Vassilios Morellas (research director), David J Mulla, Nikos Papanikolopoulos (professor)
Abstract
A major component of an efficient farming strategy is the precise detection and characterization of plant deficiencies followed by the proper deployment of fertilizers. Through the thoughtful utilization of modern computer vision techniques, it is possible to achieve positive financial and environmental results for these tasks. This work introduces an automation framework that attempts to address the three main drawbacks of existing approaches: 1) lack of generality (methods are tuned for specific data sets); 2) difficulty to apply in variable field conditions; and 3) lack of tool sophistication that limits their applicability. The cultivation of corn lies in the core of the American and global economy with 81.7 million acres harvested only in the USA for the year 2018. The ubiquity of its cultivation makes it an ideal candidate to highlight the large economic benefits from even a small improvement in nutrient deficiency detection. The proposed methodology utilizes drone collected images to detect nitrogen (N) deficiencies in maize fields and assess their severity using low-cost RGB sensors. The proposed methodology is twofold. A low complexity recommendation scheme identifies candidate plants exhibiting N deficiency and, with minimal interaction, assists the annotator in the creation of a training data set that is then used to train an object detection deep neural network. Results on data from experimental fields support the merits of the proposed methodology with mean average precision for the detection of N-deficient leaves reaching 82.3%.
Link to full paper
Keywords
computer vision, remote sensing, precision agriculture