Mining Novel Multivariate Relationships in Time Series Data Using Correlation Networks [journal]
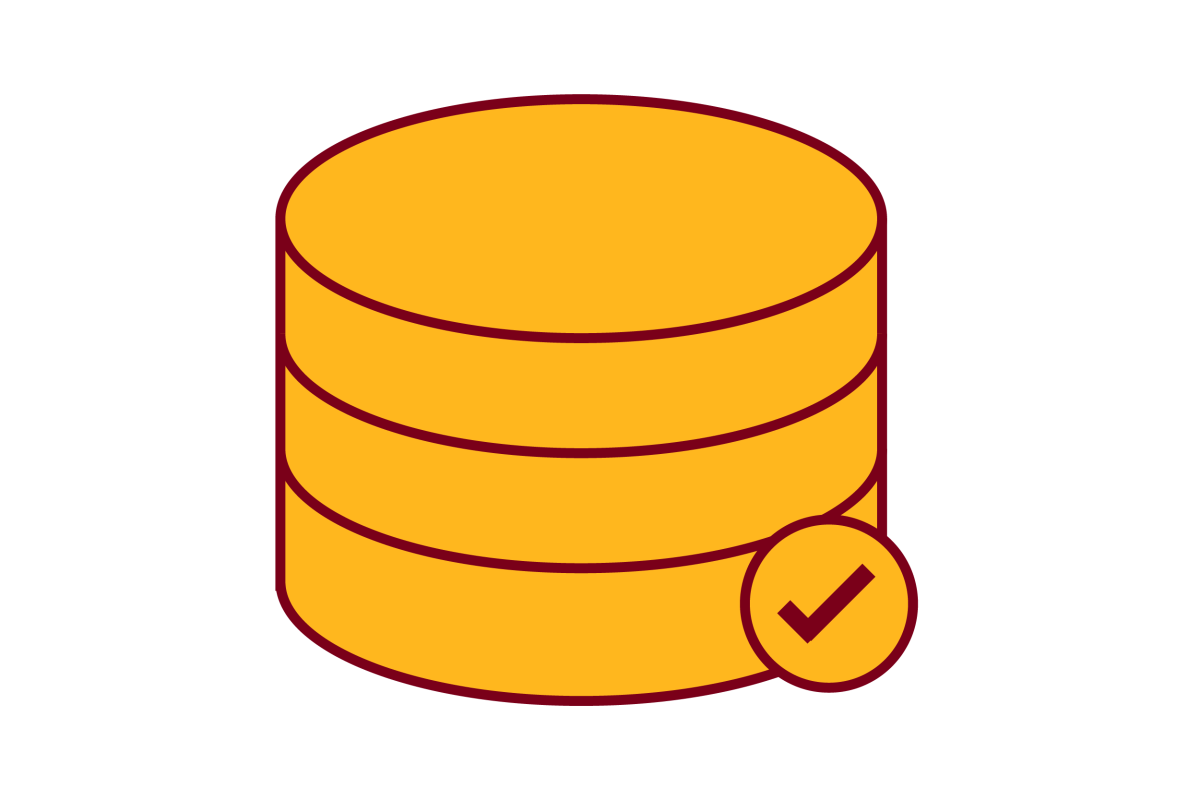
Journal
IEEE Transactions on Knowledge and Data Engineering (Volume: 32, Issue: 9, September 1, 2020)
Authors
Saurabh Agrawal (Ph.D. 2018), Michael Steinbach (researcher), Daniel Boley (professor), Snigdhansu Chatterjee, Gowtham Atluri, Anh The Dang, Stefan Liess, Vipin Kumar (professor)
Abstract
In many domains, there is significant interest in capturing novel relationships between time series that represent activities recorded at different nodes of a highly complex system. In this paper, we introduce multipoles, a novel class of linear relationships between more than two time series. A multipole is a set of time series that have strong linear dependence among themselves, with the requirement that each time series makes a significant contribution to the linear dependence. We demonstrate that most interesting multipoles can be identified as cliques of negative correlations in a correlation network. Such cliques are typically rare in a real-world correlation network, which allows us to find almost all multipoles efficiently using a clique-enumeration approach. Using our proposed framework, we demonstrate the utility of multipoles in discovering new physical phenomena in two scientific domains: climate science and neuroscience. In particular, we discovered several multipole relationships that are reproducible in multiple other independent datasets and lead to novel domain insights.
Link to full paper
Mining Novel Multivariate Relationships in Time Series Data Using Correlation Networks
Keywords
data mining