Process Guided Deep Learning for Modeling Physical Systems: An Application in Lake Temperature Modeling [conference paper]
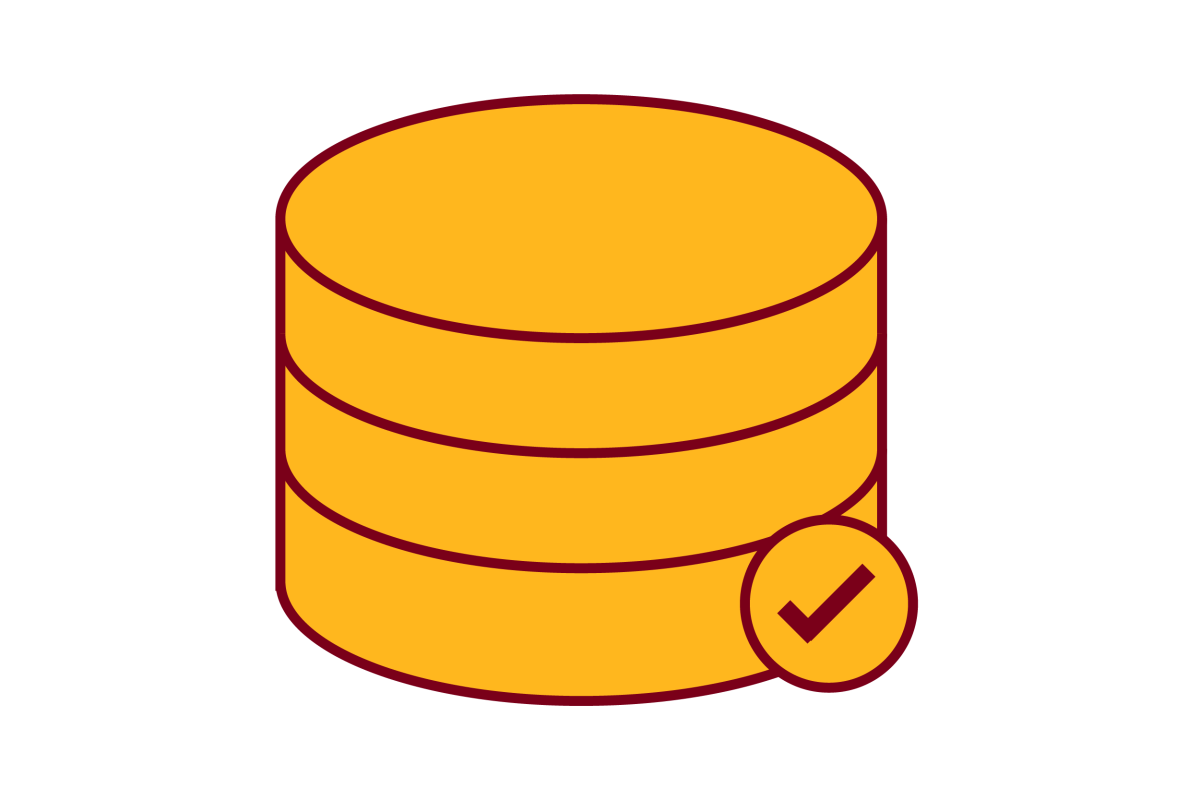
Conference
IEEE International Geoscience and Remote Sensing Symposium (IGARSS) - September 26-October 2, 2020
Authors
Xiaowei Jia (Ph.D. 2020), Jared Willard (Ph.D. student), Anuj Karpatne (Ph.D. 2017), Jordan S Read, Jacob A Zwart, Michael Steinbach (researcher), Vipin Kumar (professor)
Abstract
In this paper, we introduce a new paradigm that combines scientific knowledge within process-based models and machine learning models to advance scientific discovery in many physical systems. We will describe how to incorporate physical knowledge in real-world dynamical systems as additional constraints for training machine learning models and how to leverage the hidden knowledge encoded by existing process-based models. We evaluate this approach on modeling lake water temperature and demonstrate its superior performance using limited training data and the improved generalizability to different scenarios.
Link to full paper
Keywords
deep learning, environmental data science