Significant spatial co-distribution pattern discovery [journal]
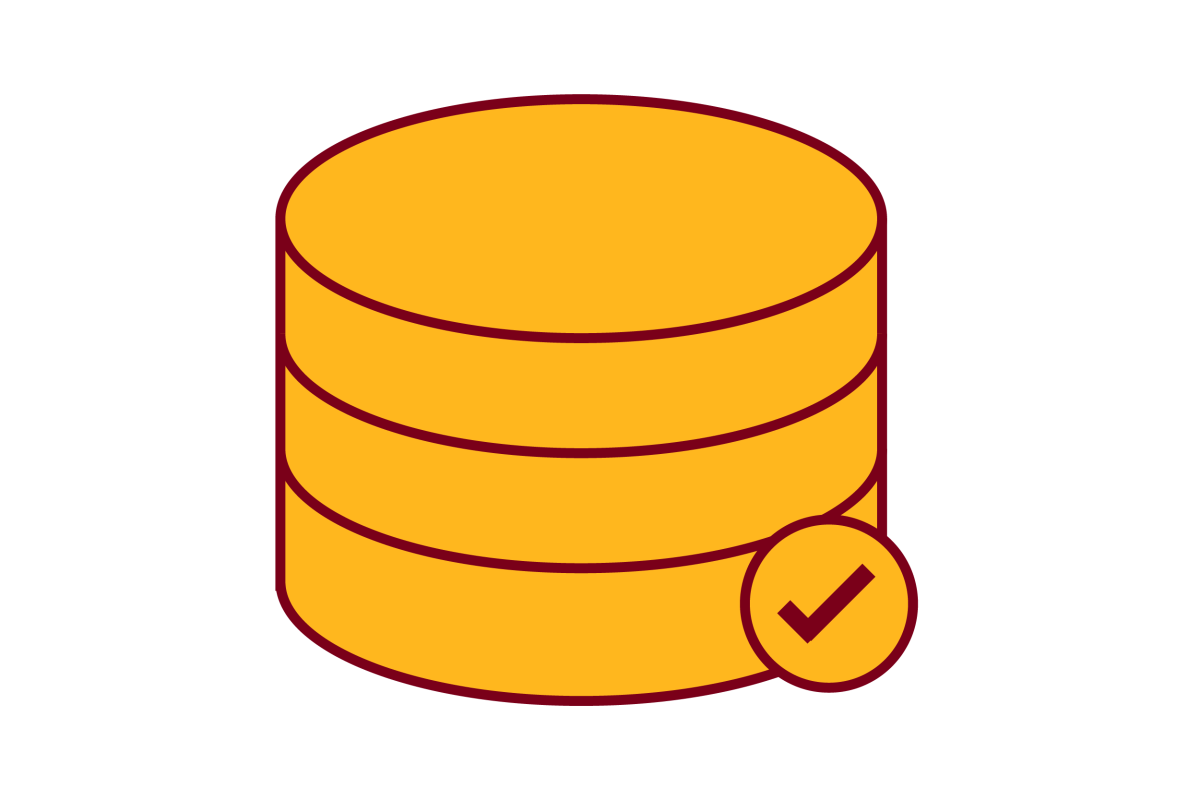
Journal
Computers, Environment and Urban Systems - November 1, 2020
Authors
Jiannan Cai (visiting Ph.D. student), Yiqun Xie (Ph.D. student), Min Deng, Xun Tang (Ph.D. student), Yan Li (Ph.D. student), Shashi Shekhar (professor)
Abstract
Given instances (spatial points) of different spatial features (categories), significant spatial co-distribution pattern discovery aims to find subsets of spatial features whose spatial distributions are statistically significantly similar to each other. Discovering significant spatial co-distribution patterns is important for many application domains such as identifying spatial associations between diseases and risk factors in spatial epidemiology. Previous methods mostly associated spatial features whose instances are frequently located together; however, this does not necessarily indicate a similarity in the spatial distributions between different features. Thus, this paper defines the significant spatial co-distribution pattern discovery problem and subsequently develops a novel method to solve it effectively. First, we propose a new measure, dissimilarity index, to quantify the difference between spatial distributions of different features under the spatial neighbor relation and then employ it in a distribution clustering method to detect candidate spatial co-distribution patterns. To further remove spurious patterns that occur accidentally, the validity of each candidate spatial co-distribution pattern is verified through a significance test under the null hypothesis that spatial distributions of different features are independent of each other. To model the null hypothesis, a distribution shift-correction method is presented by randomizing the relationships between different features and maintaining spatial structure of each feature (e.g., spatial auto-correlation). Comparisons with baseline methods using synthetic datasets demonstrate the effectiveness of the proposed method. A case study identifying co-morbidities in central Colorado is also presented to illustrate the real-world applicability of the proposed method.
Link to full paper
Significant spatial co-distribution pattern discovery
Keywords
spatial computing, data mining