Towards Spatial Variability Aware Deep Neural Networks (SVANN): A Summary of Results [preprint]
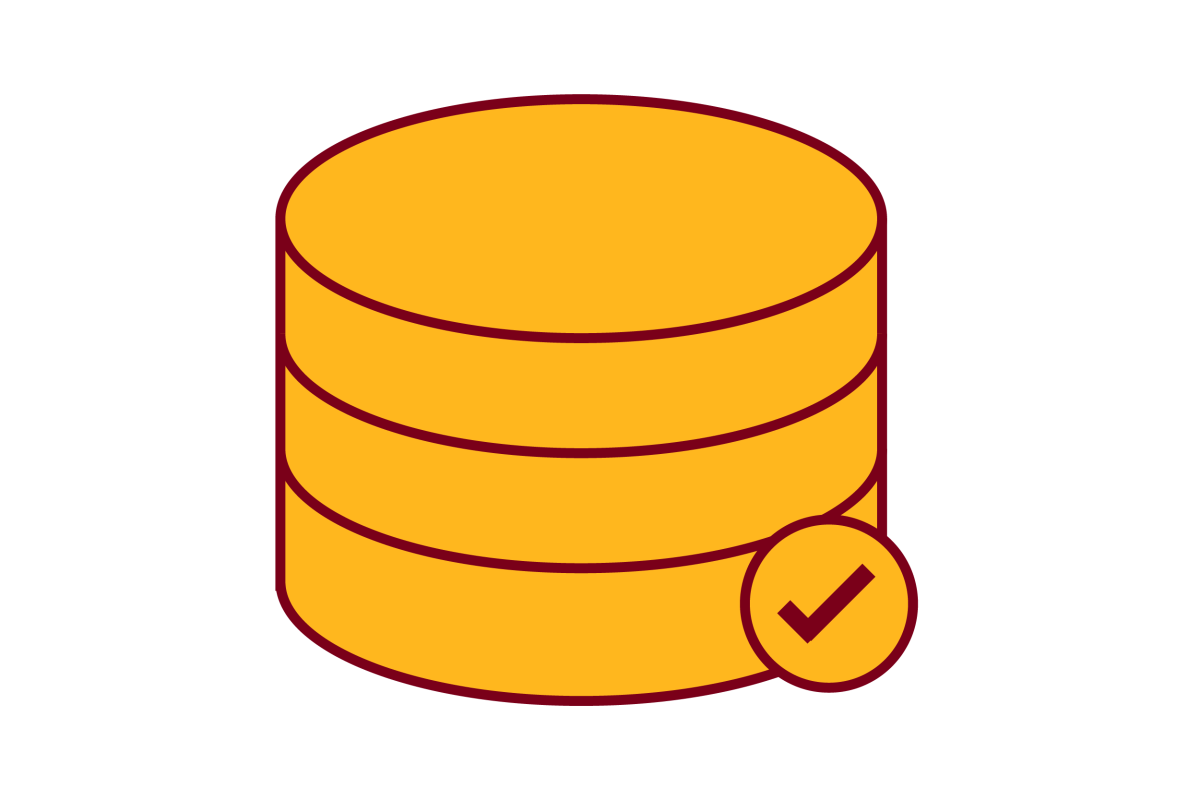
Preprint date
November 17, 2020
Authors
Jayant Gupta (Ph.D. student), Yiqun Xie (Ph.D. student), Shashi Shekhar (professor)
Abstract
Spatial variability has been observed in many geo-phenomena including climatic zones, USDA plant hardiness zones, and terrestrial habitat types (eg, forest, grasslands, wetlands, and deserts). However, current deep learning methods follow a spatial-one-size-fits-all (OSFA) approach to train single deep neural network models that do not account for spatial variability. In this work, we propose and investigate a spatial-variability aware deep neural network (SVANN) approach, where distinct deep neural network models are built for each geographic area. We evaluate this approach using aerial imagery from two geographic areas for the task of mapping urban gardens. The experimental results show that SVANN provides better performance than OSFA in terms of precision, recall, and F1-score to identify urban gardens.
Link to full paper
Towards Spatial Variability Aware Deep Neural Networks (SVANN): A Summary of Results
Keywords
spatial computing