Weakly Supervised Classification Using Group-Level Labels [preprint]
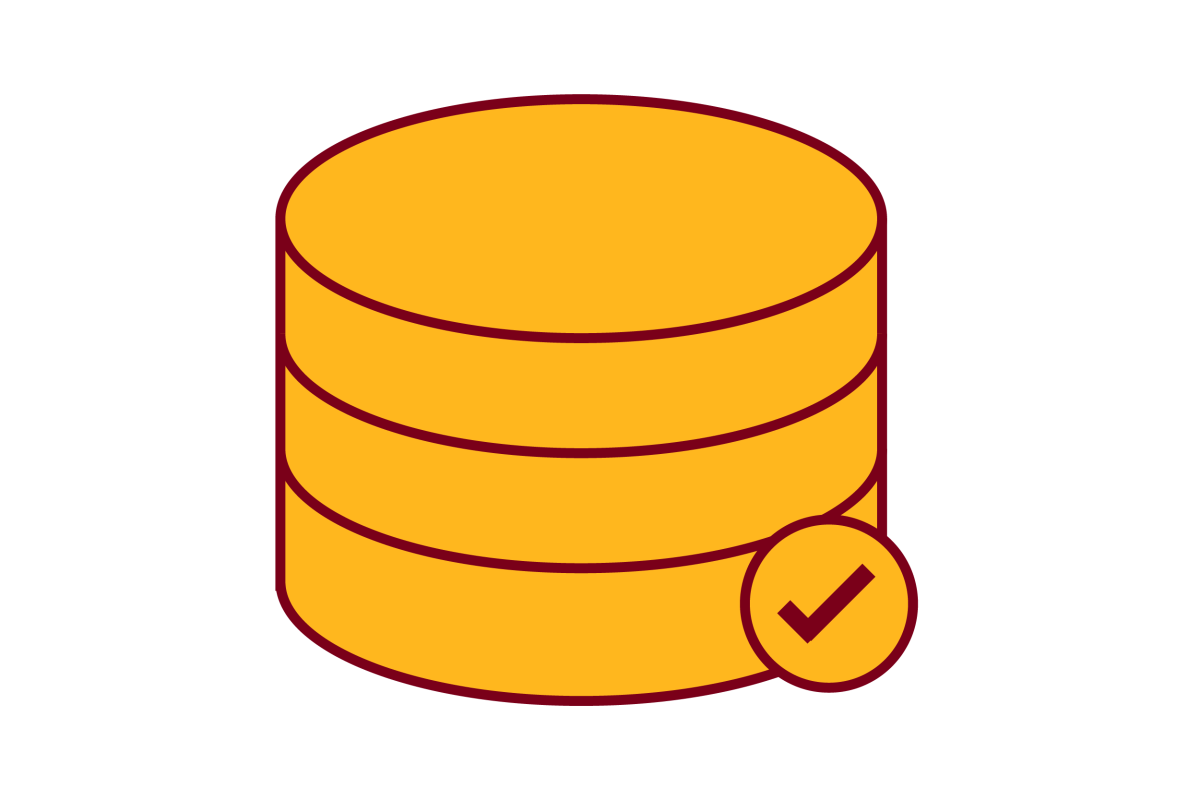
Preprint date
August 16, 2021
Authors
Guruprasad Nayak (Ph.D. 2020), Rahul Ghosh (Ph.D. student), Xiaowei Jia (Ph.D. 2020), Vipin Kumar (professor)
Abstract
In many applications, finding adequate labeled data to train predictive models is a major challenge. In this work, we propose methods to use group-level binary labels as weak supervision to train instance-level binary classification models. Aggregate labels are common in several domains where annotating on a group-level might be cheaper or might be the only way to provide annotated data without infringing on privacy. We model group-level labels as Class Conditional Noisy (CCN) labels for individual instances and use the noisy labels to regularize predictions of the model trained on the strongly-labeled instances. Our experiments on real-world application of land cover mapping shows the utility of the proposed method in leveraging group-level labels, both in the presence and absence of class imbalance.
Link to full paper
Weakly Supervised Classification Using Group-Level Labels
Keywords
machine learning